Sahyadri Conservation Series - 53 |
ENVIS Technical Report: 100, April 2016 |
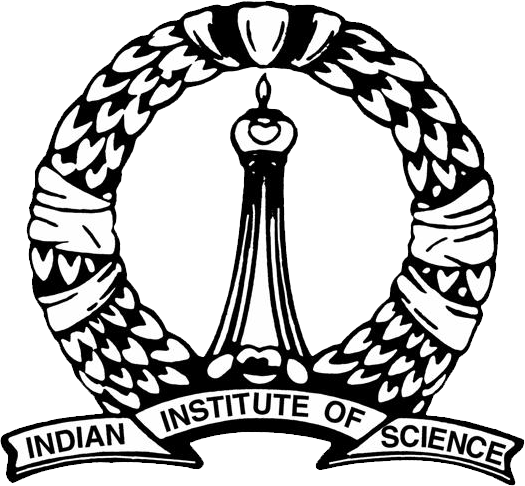 |
Time-series MODIS NDVI based Vegetation Change Analysis with Land
Surface Temperature and Rainfall in Western Ghats, India
| 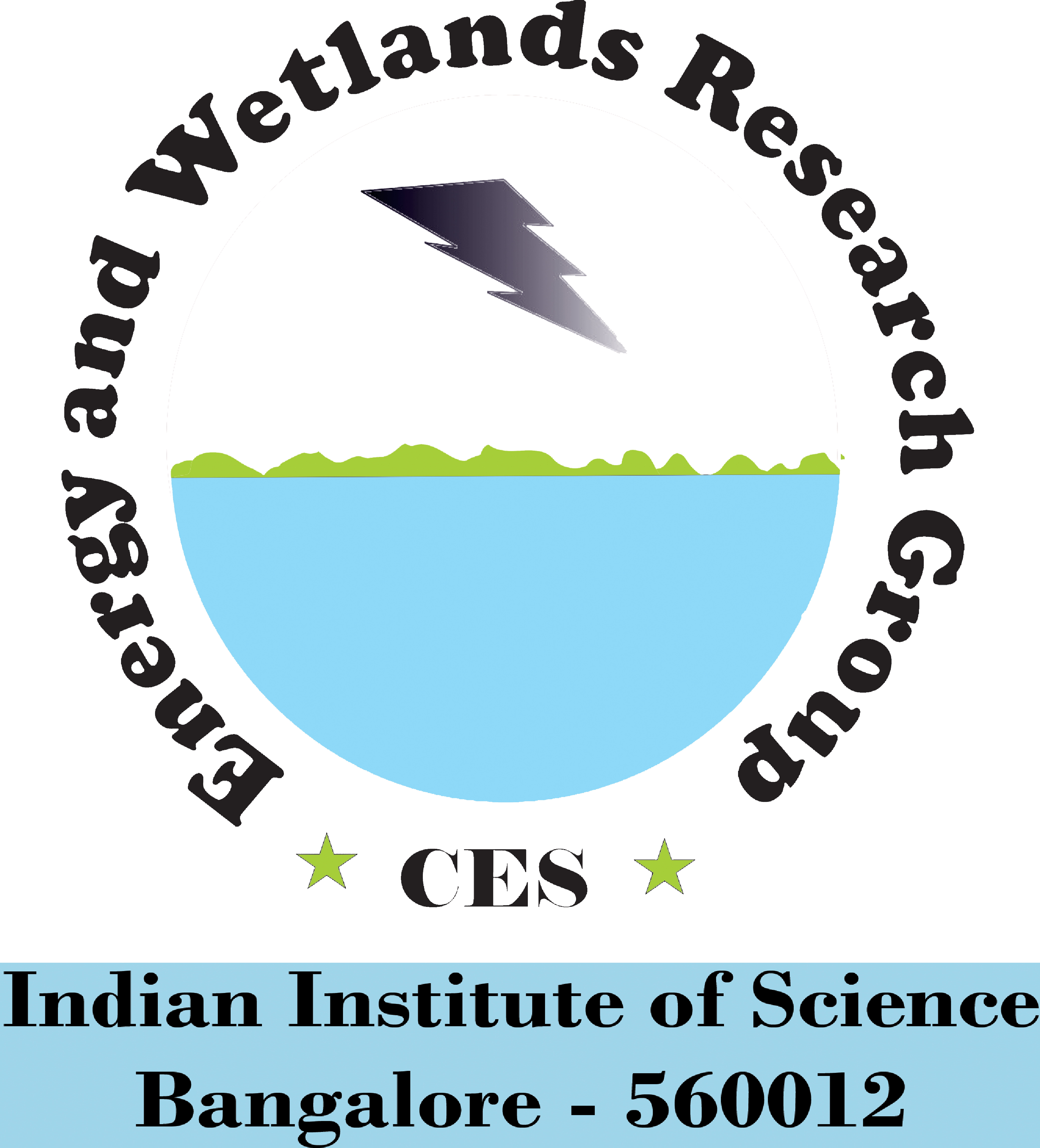
|
Vegetation plays an important role in moderating/regulating climate through the exchange of energy, water vapour and momentum between the land surface and the atmosphere (Wang, 2006). Vegetation also controls atmospheric CO2 and currently absorbs about one-third of anthropogenic fossil fuel emissions to the atmosphere. The magnitude of the gross flux of carbon taken up annually by photosynthesis is about 15 times the absolute value of fossil fuel emissions (Wang et al., 2011). Thus, even a small change in vegetation photosynthesis could have a large effect on the role of vegetation as a carbon sink. Therefore, understanding how vegetation growth responds to climate change is a critical requirement for projecting future ecosystem dynamics.
NDVI has been effectively used in vegetation dynamics monitoring and the study of vegetation responses to climatic change at different scales during the past few years (e.g., Beck et al., 2006; Tucker et al., 2001; Wang et al., 2003; Zhou et al., 2001). Using RS NDVI data to investigate vegetation changes and relationships between vegetation and climate has acquired abundant achievements.
In this study, usage of time-series NDVI was demonstrated by first deriving LC classes by thresholding method and boxplot analysis. Methods incorporated in this work included the application of an automated MODIS NDVI time-series and reference database for the extraction of LC classes (dense vegetation, agriculture/grassland, soil/settlement and water) to support multi-temporal data analysis based on ground information. These LC maps were then validated using test data collected from ground and by Google Earth time-series images. Also, significant difficulties in evaluating change detection results hold from the inability to adequately characterize outcome accuracies (Khorram et al., 1999). Unlike typical LC classification assessment that requires only single date validation data, change detection validation required data for multiple dates to provide sufficient change (i.e., before and after event) documentation. In particular, the characterization of change omission errors represents an especially difficult challenge (Lunetta et al., 2002b, 2004). Because LC change (conversion) is a relative occurrence over a large area, it is difficult to derive a robust estimate of change omission errors.
Although MODIS NDVI data for the month of July was not considered in our analysis because of the presence of cloud, MODIS NDVI data processing was conducted to provide a filtered and cleaned (anomalous data removed) uninterrupted data stream to support multi-temporal (phenological) analysis. Total annual NDVI values for each 250 m grid cell within the study area (2003–2012) were compared on an annual basis to identify those cells exhibiting greater than specified threshold values and were labeled as LC conversion areas. Intensive LC activities associated with agricultural crop rotations often confound LC conversion determinations. To summarize, table 19 shows decadal changes in area of LC in Western Ghats. Table 20 shows changes in LST and table 21 highlights the decadal pattern in rainfall.
Table 19: Decadal changes in area of LC in Western Ghats
Class |
2003 |
2012 |
Decadal changes |
ha |
% |
Ha |
% |
ha |
% |
Northern Western Ghats |
|
Dense forest |
2340719 |
23.64 |
2059516 |
20.80 |
281203 |
2.84 |
↓ |
Agriculture/ grassland |
7315449 |
73.88 |
7536259 |
76.11 |
220810 |
2.23 |
↑ |
Settlement/ soil |
108282.6 |
1.09 |
182789 |
1.84 |
74506.4 |
0.75 |
↑ |
Water |
138085.2 |
1.39 |
123183.9 |
1.24 |
14901 |
0.15 |
↓ |
Central Western Ghats |
|
Dense forest |
5073131 |
53.39 |
4656546 |
49.01 |
416585 |
4.38 |
↓ |
Agriculture/ grassland |
4331023 |
45.58 |
4741510 |
49.9 |
410487 |
4.32 |
↑ |
Settlement/ soil |
4751 |
0.05 |
49410.4 |
0.52 |
44659.4 |
0.47 |
↑ |
Water |
93119.6 |
0.98 |
56061.8 |
0.59 |
37057.8 |
0.39 |
↓ |
Southern Western Ghats |
|
Dense forest |
5897318 |
78.78 |
5465387 |
73.01 |
431931 |
5.77 |
↓ |
Agriculture/ grassland |
1538333 |
20.55 |
1976253 |
26.4 |
437920 |
5.85 |
↑ |
Settlement/ soil |
7485.81 |
0.1 |
8982.97 |
0.12 |
1497.16 |
0.02 |
↑ |
Water |
42669.09 |
0.57 |
35183.29 |
0.47 |
7485.8 |
0.1 |
↓ |
Table 20: Decadal changes in LST in Western Ghats
Class |
LST (°C) ± sd (2003) |
LST (°C) ± sd (2012) |
summer |
monsoon |
Winter |
summer |
monsoon |
winter |
Northern Western Ghats |
Dense forest |
28±4 |
26±2 |
21±3 |
29±3 |
25±2 |
19±3 |
Agriculture/ grassland |
33±4 |
29±2 |
26±3 |
33±3 |
29±3 |
24±4 |
Central Western Ghats |
Dense forest |
28±3 |
24±2 |
19±4 |
26±3 |
24±2 |
19±4 |
Agriculture/ grassland |
33±3 |
27±3 |
26±4 |
31±3 |
27±3 |
26±4 |
Southern Western Ghats |
Dense forest |
28±2 |
27±1 |
26±2 |
29±2 |
28±1 |
27±3 |
Agriculture/ grassland |
29±2 |
28±2 |
29±1 |
28±3 |
29±2 |
31±2 |
Table 21: Decadal changes in rainfall in Western Ghats
Class |
2003 |
2012 |
Total rainfall (mm) |
Total rainfall (mm) |
Northern Western Ghats |
Dense forest |
241.51 |
330.55 |
Agriculture/grassland |
262.81 |
276.43 |
Central Western Ghats |
Dense forest |
248.26 |
431.55 |
Agriculture/grassland |
170.67 |
338.49 |
Southern Western Ghats |
Dense forest |
276.27 |
503.98 |
Agriculture/grassland |
240.2 |
432.61 |
Table 19 shows that dense forest area has considerably decreased by 2.84% (281203 ha), 4.38% (416585 ha), and 5.77% (431931 ha), and agricultural/grassland area has increased by 2.23% (220810 ha), 4.32% (410487 ha) and 5.85% (437920 ha) in the northern, central and southern Western Ghats. There is a marginal increase in settlement/soil area and small decrease in the spatial extent of water bodies in all the three regions (see table 19). Table 20 reveals that temperature in dense forest has always been lesser than in agricultural/grassland areas in all the three seasons. Temperature has a decreasing trend from summer to monsoon to winter except in southern Western Ghats, where winter temperatures were higher. Overall, there has not been much variation in the seasonal temperature during the study period. Total annual rainfall had an increasing trend from 2003 to 2012 (table 21), but number of rainy days have decreased in southern Western Ghats.
Joint analysis of NDVI and LST showed to be of great significance, since they facilitate the identification of changes in land occupation and surface conditions (Nemani et al., 1993) by differentiating seasonal changes from changes in land occupation (Nemani and Running, 1997). NDVI and LST behaviors have also been proven to be partially correlated (Kaufmann et al., 2003): an increase in NDVI values during summer (greater proportion of vegetation) results in lower LST, while higher NDVI values during winter results in an increase in LST.
Correlation analyses between NDVI and climate variable is a powerful tool for probing ecosystem function response to climate change. The present study combined datasets of NDVI from 2003 to 2012 and climate parameters to analyze year to year variations. In this work we described the spatial relationship between MODIS NDVI, MODIS derived LST and rainfall. We focused on vegetation data to investigate the distribution of LST and rainfall because the existing vegetation distribution is largely controlled by temperature and precipitation pattern. Monthly climatic changes and trends in the 10 years provided a clear illustration of the NDVI trends. Differences in NDVI–temperature and NDVI–precipitation correlations relative to vegetation types such as forest and agriculture/grassland were also comprehensively investigated. This result supports and is of similar magnitude as temporal studies showing increase of NDVI corresponding to increase in growing season temperature over the length of the satellite record. The main outcomes from this study can be summarized in the following points.
- Northern and central Western Ghats showed very similar trends. Dense vegetation/forest area increased in September–October–November and decreased in January–February. This season (August–September–October) corresponds from mid to end of the monsoon that brings heavy showers from the Arabian Sea. Due to this rain, entire Ghat section is full of thick vegetation.
- Agriculture/grassland area is maximum in August–September and December–January and minimum in April–May in both northern and central Western Ghats.
- Southern Western Ghats shows a slightly different pattern with peak dense vegetation between October through April and lowest in August and September. Agriculture crops occupy maximum spatial extent between August to December and shows valleys in the time-series graph between April to June.
- The total dense green area in northern and central Western Ghats are between 30– 70% of all the LC classes while dense vegetation constitute around 60–90% of the southern Western Ghats throughout the years of study (2003–2012).
- NDVI values for water tend to increase whenever there is a peak in vegetation across the months and year (in monsoon season) in all the three regions of Western Ghats.
- Image to image correlation coefficient (at 0.01 significance level) between NDVI of forest with LST and rainfall, and NDVI of agriculture/grassland class with LST and rainfall for northern, central and southern Western Ghats were computed that reconfirmed the negative correlation between NDVI and LST in northern and central Western Ghats, and negative to weak positive correlation in southern Western Ghats. Most months showed weak negative to strong positive correlation between NDVI and rainfall during 2003–2012 in northern and central Western Ghats. Southern Western Ghats exhibited strong negative to weak positive correlation between 2003 to 2008 and weak negative to stronger positive correlation between NDVI and rainfall from 2009–12. Correlation between NDVI–LST decreased and NDVI–rainfall increased as one moves from forest to grassland.
- The seasonal analysis revealed that mean NDVI of forest is highest in southern Western Ghats followed by central Western Ghats and least in northern Western Ghats. Mean LST is lowest is central Western Ghats followed by northern Western Ghats. In southern Western Ghats, LST is almost constant (around 27 °C) with least deviation. Total rainfall is minimum in northern Western Ghats and maximum in southern Western Ghats.
- In northern Western Ghats, NDVI and LST show increasing trend and rainfall has a decreasing trend. In summer, rainfall is minimum and static from 2009 onwards. Monsoon temperature increased from 2010 onwards indicating more photosynthetic activity and increased NDVI. Winter temperature has increased and rainfall has decreased but have the maximum NDVI during this season.
- In central Western Ghats, summers are dry and NDVI follows a cyclic pattern with both temperature and rainfall following a decreasing trend. Monsoon has higher LST and rainfall which triggers growing season environment for dense vegetation. Winters are warmer with increasing rainfall trend in the decade.
- Southern Western Ghats has overall higher NDVI, LST and total rainfall among all seasons in the entire Western Ghats. Summer and winter LST have increased, NDVI have been decreasing and rainfall trend has decreased in summer and increased in monsoon and winter during 2003 to 2012.
- Lower NDVI values were observed during March to June and high NDVI values were seen during August to January/February for all the years for both dense vegetation and agriculture in northern Western Ghats.
- In central Western Ghats, NDVI values for green vegetation is higher, the minimum-maximum temperature range is lower i.e. the regions is cooler and the area witnesses higher rainfall compared to northern Western Ghats.
- Southern Western Ghats exhibit higher NDVI values throughout the year for dense vegetation (> 0.55) with maximum NDVI reaching 0.79. The mean temperature variation is very small (~23 to ~35 °C) throughout the year and rainfall is more during April to October/November.
- Rainfall time-series data in forest and agricultural/grassland areas were modelled and forecasted using ARIMA which indicated a decreasing trend in the rainfall pattern over forest and agricultural/grassland from 2013 towards 2020 in northern, central and southern Western Ghats.
There are also, however, several limitations of deriving LC and phenological information from NDVI. Uncertainties in relating NDVI to vegetation are associated with the effects of atmospheric variations, sensor calibration, and sensor degradation over time (James and Kullari, 1994; Townshend, 1995). Surface heterogeneity also complicates interpretation of NDVI. Due to low NDVI values that result from sparse vegetation as in northern Western Ghats, uncertainties in interpreting NDVI can sometimes increase. Vegetation canopies in such environments do not achieve complete coverage, making NDVI susceptible to the spectral influence of the soil and soil moisture in gaps between vegetation (Peters and Eve, 1995).
This study provided not only the current LC status of the Western Ghats, but also identified both the pattern and nature of changes that have impacted the local temperature and rainfall pattern. The analysis highlighted and confirmed several important MODIS data applications, such as a) potentially substantial cost saving on data procurement and analysis, b) minimization of image registration errors that would have otherwise occurred while using multisensor data that typically limit the overlay of multiple date coverage (post-classification) to support change detection analysis, c) practical methods for large area change detection monitoring (using MODIS data) including significant reduction of inter and intraannual vegetation phenology mediated errors and their assessment.
Though this NDVI based analysis of change in vegetation is a significant contribution to the previous researches conducted in this region, future studies could improve the understanding of climate change effects on vegetation growth in a longer time-series. Future studies will involve the use of HANTS (Harmonic ANalysis of Time Series) algorithm to perform the detection of cloud contaminated pixels. Hants algorithm allows the simultaneous observation of mean value, first harmonic amplitude and phase behaviors in the same image (Julien et al., 2006). New studies can combine process-based ecosystem models and climate models and explore how vegetation growth changes affect the terrestrial carbon cycle and its feedback to the Earth's climate system. This study would undoubtedly help to identify the main factors influencing spatio-temporal patterns in vegetation growth over 10 years.
T.V. Ramachandra
Centre for Sustainable Technologies, Centre for infrastructure, Sustainable Transportation and Urban Planning (CiSTUP), Energy & Wetlands Research Group, Centre for Ecological Sciences, Indian Institute of Science, Bangalore – 560 012, INDIA.
E-mail : cestvr@ces.iisc.ernet.in
Tel:91-080-22933099/23600985,
Fax:91-080-23601428/23600085
Web: http://ces.iisc.ernet.in/energy
Uttam Kumar
Energy and Wetlands Research Group, Centre for Ecological Sciences. Indian Institute of Science, Bangalore – 560 012, India
E-mail: uttam@ces.iisc.ernet.in
Anindita Dasgupta Energy and Wetlands Research Group, Centre for Ecological Sciences. Indian Institute of Science, Bangalore – 560 012, India
Bharath Setturu Energy and Wetlands Research Group, Centre for Ecological Sciences. Indian Institute of Science, Bangalore – 560 012, India
E-mail: settur@ces.iisc.ernet.in
Vinay S Energy and Wetlands Research Group, Centre for Ecological Sciences. Indian Institute of Science, Bangalore – 560 012, India
E-mail: vinay@ces.iisc.ernet.in
Harish R Bhat Energy and Wetlands Research Group, Centre for Ecological Sciences. Indian Institute of Science, Bangalore – 560 012, India
E-mail: harishrbhat@ces.iisc.ernet.in
Citation: Ramachandra T V, Uttam Kumar and Anindita Dasgupta, 2016, Time-series MODIS NDVI based Vegetation Change Analysis with Land Surface Temperature and Rainfall in Western Ghats, India, ENVIS Technical Report 100, Sahyadri Conservation Series 53, Energy & Wetlands Research Group, CES, Indian Institute of Science, Bangalore 560012.
|