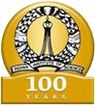 |
|
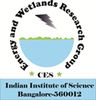 |
Conclusions
The utility of MLP and DT in classifying MODIS data is compared in this communication. The results showed that MLP on MNF components was best for LC mapping (86% accuracy) at regional scale. This technique was able to map agriculture, forest and water bodies properly, whereas DT is good in classifying built up areas, plantation and waste land. The results from this study can lead to better mapping of various LC features from MODIS data with the help of ancillary data (secondary data) in the absence of high resolution imagery. One potential limitation of the study is that the MODIS pixels could as well be softly classified using unmixing techniques (such as Linear unmixing, or NN based unmixing) rather than using hard classification that assigns a single class to each pixel. The utility of these unmixing techniques then would have been studied on different MODIS products. The unmixing techniques give a better estimate of the percentage area of different land cover classes since most landscapes are heterogeneous in nature and are a mixture of various land cover classes. This could be the future work of the current study.
Citation: Uttam Kumar, Norman Kerle, Milap Punia and T. V. Ramachandra , 2011, Mining Land Cover Information Using Multilayer. J Indian Soc Remote Sens,
DOI 10.1007/s12524-011-0061-y.
U. Kumar
Department of Management Studies and Centre
for Sustainable Technologies, Indian Institute of Science,
Bangalore 560012, India
e-mail: uttam@ces.iisc.ernet.in |
|
M. Punia
Centre for the Study of Regional Development,
Jawaharlal Nehru University,
New Delhi 110067, India
e-mail: m_punia@hotmail.com |
N. Kerle
Department of Earth Systems Analysis,
Faculty of Geo-Information Science and Earth Observation,
Twente University,
P.O. Box. 6, 7500 AA Enschede, The Netherlands
e-mail: kerle@itc.nl |
|
T. V. Ramachandra (*)
Centre for Ecological Sciences and Centre for Sustainable
Technologies, Indian Institute of Science,
Bangalore 560012, India
phone: 91-80-22933099; fax: 91-80-23601428; e-mail: cestvr@ces.iisc.ernet.in |
|