Results and Discussion
Precipitation of wettest month and wettest quarter of the year were used as different layers along with a set of seven other layers as mentioned earlier. Fig. 6 (a) and (b) indicate the landslide probability maps using GARP and SVM on precipitation of wettest month. The landslide occurrence points were overlaid on the probability maps to validate the prediction as shown in fig. 7. The GARP map had an accuracy of 92% and SVM map was 96% accurate with respect to the ground and Kappa values 0.8733 and 0.9083 respectively. The corresponding ROC curves are shown in fig. 8 (a) and (b). Total area under curve (AUC) for fig. 8 (a) is 0.87 and for fig. 8 (b) is 0.93. Figure. 6 (c) and (d) are the landslide probability maps using GARP and SVM on precipitation of wettest quarter with accuracy of 91% and 94% and Kappa values of 0.9014 and 0.9387 respectively. ROC curves in figure 8 (c) and (d) show AUC as 0.90 and 0.94.
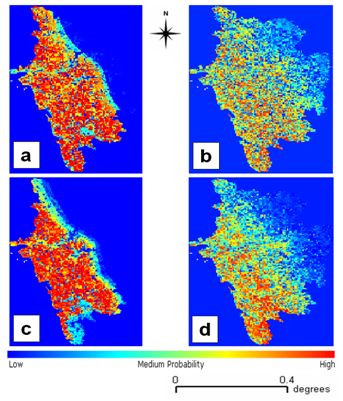
Fig. 6: Probability distribution of the landslide prone areas
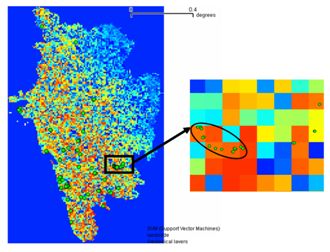
Fig. 7: Validation of the probability distribution of the landslide prone areas by overlaying landslide occurrence points
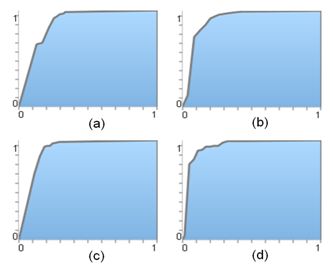
Fig. 8: ROC curves for landslide prone maps (a) GARP (b) SVM on precipitation of wettest month; (c) GARP and (d) SVM on
Various measures of accuracy were used as per Fielding and Bell to assess the outputs. Table I presents the confusion matrix structure indicating true positives, false positives, false negatives and true negatives. Confusion matrices were generated for each of the 4 outputs (Table II) and different measures of accuracy such as prevalence, global diagnostic power, correct classification rate, sensitivity, specificity omission and commission error computed and listed as in table III.
Table 1: Confusion Matrix
|
True Presence |
True Absence |
Predicted Presence |
A |
B |
Predicted Absence |
C |
D |
Key: A – True Positive, B – False Positive, C – False Negative, D – True Negative
Table 2: Confusion matrix for GARP and SVM Outputs for Uttara Kannada
Uttara kannada |
True presence |
True absence |
Number of usable presence |
Number of usable absence |
GARP with precipitation of wettest month |
Predicted Presence |
120 |
0 |
125 |
0 |
Predicted Absence |
5 |
0 |
SVM with precipitation of wettest month |
Predicted Presence |
118 |
0 |
125 |
0 |
Predicted Presence |
7 |
0 |
GARP with precipitation of wettest Quarter |
Predicted Presence |
118 |
0 |
125 |
0 |
Predicted Presence |
7 |
0 |
SVM with precipitation of wettest Quarter |
Predicted Presence |
117 |
0 |
125 |
0 |
Predicted Presence |
8 |
0 |
Table 3: Statistics of GARP and SVM Outputs for Uttara Kannada
Uttara Kannada |
Prevalence (A+C)/N |
Global diagnostic Power (B+D)/N |
Correct Classification Rate (A+D)/N |
Sensitivity A/(A+C) |
Specificity D/(B+D) |
Omission Error C/(A+C) |
Commision Error B/(B+D) |
GARP with precipitation of wettest month |
- |
- |
0.96 |
0.96 |
- |
0.04 |
- |
SVM with precipitation of wettest month |
- |
- |
0.94 |
0.94 |
- |
0.06 |
- |
GARP with precipitation of wettest Quarter |
- |
- |
0.94 |
0.94 |
- |
0.06 |
- |
SVM with precipitation of wettest Quarter |
- |
- |
0.94 |
0.94 |
- |
0.06 |
- |
The results indicate that the output obtained from SVM using precipitation of the wettest month was best among the 4 scenarios. It may be noted that the outputs from GARP for both the wettest precipitation month and quarter are close to the SVM in term of accuracy. One reason is that most of the areas have been predicted as probable landslide prone zones [indicated in red in fig. 6 (a) and (c)] with the highly undulating terrain consisting of steep slopes frequently exposed to landslides induced by rainfall. This was validated with the data collected from the field that showed overlap with the maximum number of landslide points occurring in the undulating terrain. This indicates that the region is more susceptible to landslides compared to north-eastern part of the district which has relatively flat terrain.
The exercise was repeated for another undulating terrain in the Western Ghats - Kerala state, India with the environmental layers along with 10 landslide occurrence field points as shown in fig. 9. The predicted output for precipitation in the wettest month is shown in fig. 10 and precipitation in the wettest quarter is shown in fig. 11 with an overall accuracy of 60%. The Kappa values for two cases were 0.966 and 0.965. The ROC curves are shown in fig. 12 (a) and (b) with 0.97 AUC for both the cases respectively. The confusion matrix and statistics are presented in table IV and V. The reason for low accuracy is due to lower field data collection (10 occurrence points) throughout the state. Also, the coarse resolution of the pixel has contributed for poor accuracy.
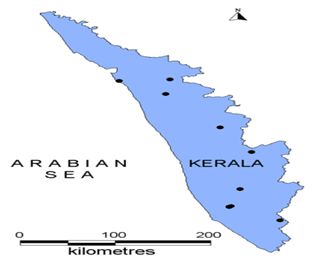
Fig. 9: Landslide occurrence points in Kerala
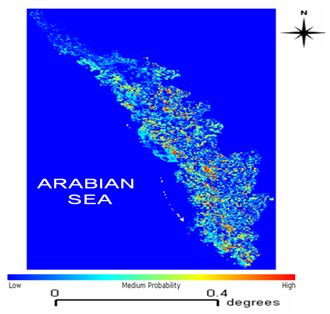
Fig. 10: Probability distribution of the landslide prone areas in Kerala using precipitation in the wettest month data
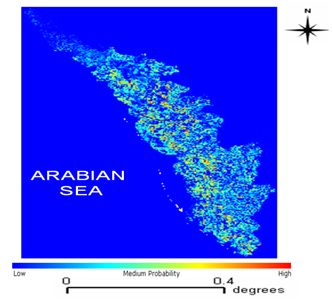
Fig. 11: Probability distribution of the landslide prone areas in Kerala using precipitation in the wettest quarter data
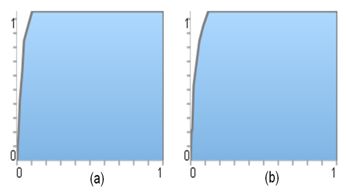
Fig. 12: ROC curves for the predicted landslide prone maps of Kerala
(a) using precipitation of the wettest month, (b) using precipitation of the wettest quarter
Table 4: Confusion matrix for GARP Outputs for Kerala
Kerala |
True presence |
True absence |
Number of usable presence |
Number of usable absence |
GARP with precipitation of wettest month |
Predicted Presence |
6 |
0 |
10 |
0 |
Predicted Absence |
4 |
0 |
GARP with precipitation of wettest Quarter |
Predicted Presence |
6 |
0 |
10 |
0 |
Predicted Presence |
4 |
0 |
Table 5: STATISTICS of GARP Outputs for Kerala
Kerala |
Prevalence (A+C)/N |
Global diagnostic Power (B+D)/N |
Correct Classification Rate (A+D)/N |
Sensitivity A/(A+C) |
Specificity D/(B+D) |
Omission Error C/(A+C) |
Commision Error B/(B+D) |
GARP with precipitation of wettest month |
- |
- |
0.6 |
0.6 |
- |
0.4 |
- |
GARP with precipitation of wettest Quarter |
- |
- |
0.6 |
0.6 |
- |
0.4 |
- |
* Key: A – True Positive, B – False Positive, C – False Negative, D – True Negative, N – Number of Samples.
However, the Kappa value and the AUC are high which indicate that the probability distribution of the predicted points falls well within the occurrence points. The intensity of these landslide points may vary from low to medium to high. One potential limitation of the above data is their spatial resolution – 1 km. It is highly unlikely that landslides of this magnitude can occur in the study area. However, given the environmental layers along with the occurrence points, the probable areas of occurrence can be mapped with great certainty.
The movement of a slope is complex and is induced or perturbed by many other factors besides rainfall such as groundwater table, soil moisture etc. which were not used in the prediction. It is known that there are accelerations of slope movements after intense rainfall, however, to what extent rainfall affects the slope movement remains unknown and the correlation between rainfall and the slope movement could be ambiguous in absence of detailed observations. Timely rainfall, soil moisture, grain-size, lithology, geological structure, seismological observations and the longer time intervals for which data are available can improve the accuracy of the model.
Beyond their key role in identifying and mapping the landslides, the choice of the variables (environmental layers) used for the prediction is also closely involved. Results can be improved with additional supplementary data such as hydrological field data etc. However, the results obtained in the current study are significant as most influential variables of landslide were used.
Natural disasters have drastically increased over the last decades. National, state and local government including NGOs are concerned with the loss of human life and damage to property caused by natural disasters. The trend of increasing incidences of landslides occurrence is expected to continue in the next decades due to unplanned anthropogenic activities leading to large scale land cover changes, deforestation and increased regional precipitation in landslide-prone areas due to changing climatic patterns47. Modern technologies with prediction ability help to mitigate the effects of natural hazards including landslides. In fact, to ensure hazard free in mountainous regions, the development of urban facilities such as homes and new roads must consider geological and geotechnical factors. Therefore, it is desired to have a notion about the main factors controlling the slope instability, assessing its severity, discriminating areas with presence/absence of landslides, updating and interpreting landslide data and determining areas that are prone to landslides.
|