LIFE CYCLE ASSESSMENT (LCA) IN AGRO-RESIDUE BIOENERGY
Considering the environmental uncertainties associated with bioenergy production as discussed in the Introduction part, it is imperative to analyze the pros and cons of bioenergy generation from a Life Cycle Assessment (LCA) prospective. The LCA is a tool to define the environmental burdens from a product, process or activity by identifying and quantifying energy and materials usage, as well as waste discharges, assessing the impacts of these wastes on the environment and it also evaluates the opportunities for environmental improvements over the whole life cycle (Singh and Olsen, 2012). LCA also helps streamline the production process by suggesting the best alternatives to minimize the overall envi- ronmental impact. There are four main steps in LCA study: (a) goal and scope definition, where the goal and scope of the study, system boundary, functional unit are identified and defined, (b) life cycle inventory (LCI), life cycle of the product under study is modelled considering all the inputs and outputs; (c) life cycle inventory anal- ysis (LCIA), where the environmental relevance of all the inputs and outputs of a product is assessed, and, (d) Interpretation, the results of the study are reported and possible measures to reduce burden on the environment is suggested at this stage. The various phases of LCA study are shown in Fig. 1 (Rathore et al., 2013). So, LCA is basically a systematic study of a product’s life cycle, primar- ily aiming at reducing its environmental burden (in other words increasing the product’s environmental acceptability).
In any bioenergy production system, the farming stage results in significant GHGs emissions and other environmental impacts due to the use of energy intensive farm machinery for irrigation, land preparation, sowing, harvest, collection and transportation of feedstock. Production and application of synthetic fertilizer and pesticide also lead to emissions and impacts soil and water quality. It is reported that, in case of sugarcane-based bioenergy generation, the farming stage has the highest environmental impacts due to land use, fuel and agro-chemicals consumption (Contreras et al., 2009). Sugarcane bagasse can be used for produc- tion of both bioelectricity and bioethanol. Lower energy related emissions could be achieved if bagasse is used for co-generation based electricity compared to bagasse bioethanol or fossil energy system (Botha and von Blottnitz, 2006; Ramjeawon, 2008). Further, integrated production of first generation (1G) and second genera- tion (2G) bagasse bioethanol has been proposed as an environmen- tally better option than 1G conventional production process (Gnansounou et al., 2015). In a comparative cradle-to-gate LCA of sugarcane bioethanol production in India and Brazil, Tsiropoulos et al. (2014) observed that Indian bioethanol causes lower or equal GHGs emissions, non-renewable energy use, human health impacts and ecosystem impairment compared to Brazilian bioethanol. The possible reason for such lower emissions is that the Indian bioethanol program is largely based on sugarcane molasses, a by-product of sugar production system, resulting in the allocation of environmental burden between product (sugar) and by-product (molasses). The cradle-to-gate LCA is a partial LCA from resource extraction (cradle) to factory gate (gate). On the other hand, the cradle-to-grave is the full LCA from resource extrac- tion (cradle) to waste disposal (grave).
LCA studies have also demonstrated the environmental benefits of rice and corn residue based bioenergy production (Shafie et al.,
2014; Sanscartier et al., 2014; Nguyen et al., 2013; Soam et al.,
2017). Soam et al. (2017) reported that electricity production from rice straw produces a higher GHGs emissions reduction compared to biogas production in Indian condition. In Malaysia also, rice straw based power generation was indicated to emit less GHGs in comparison with coal or natural gas (Shafie et al., 2014). How- ever, according to Silalertruksa and Gheewala (2013), bioethanol production from rice straw results in higher environmental benefit compared to combustion-based power production or thermo- chemical conversion of straw to Biomass-Dimethyl Ether (bio- DME). On the other hand, according to Tonini et al. (2016), biofuel production from agro-residues without involving land use change is a promising emissions reduction option, but the feed-sector’s annual crops or residues should not be used to produce biofuel, as land use change related GHGs emissions exceeds any GHGs sav- ings from displacing conventional energy sources. Using corn cob as fuel pellets for electricity generation, a 40% and 80% reduction in GHGs emissions compared to coal and natural gas combined cycle (NGCC) has been reported by Sanscartier et al. (2014). LCA study also finds that co-firing of biomass with coal results in sub- stantial emissions reduction (Sebastián et al., 2011). However, the quantity of emissions reduction will depend upon the degree of biomass pre-treatment and coal boiler efficiency and hence the use biomass with low pre-treatment and with minimum effect on boiler efficiency is suggested to maximize emissions reduction (Sebastián et al., 2011). Furthermore, the size and design parame- ters of biomass power plant also influence the emission pattern. Butnar et al. (2010) reported that, biomass power plant with gen- eration capacity in the range of 10–25 MW yield better environ- mental performance, since for bigger power plant (>25 MW), the higher efficiency of electricity production is overtaken by the higher biomass transport distance and constraints of land avail- ability for biomass cultivation. A. Singh et al. (2011), through a LCA study reported that the type of biogas reactor has an influence on emissions savings by up to 15%. Although the use of biomass (such as wheat straw, poplar and Ethiopian mustard) as a substi- tute to coal or natural gas reduces global warming, non- renewable energy use, human toxicity and eco-toxicity, however, it also leads to increased risk of eutrophication, photochemical ozone and respiratory inorganic (Nguyen et al., 2013; Kimming et al., 2011).
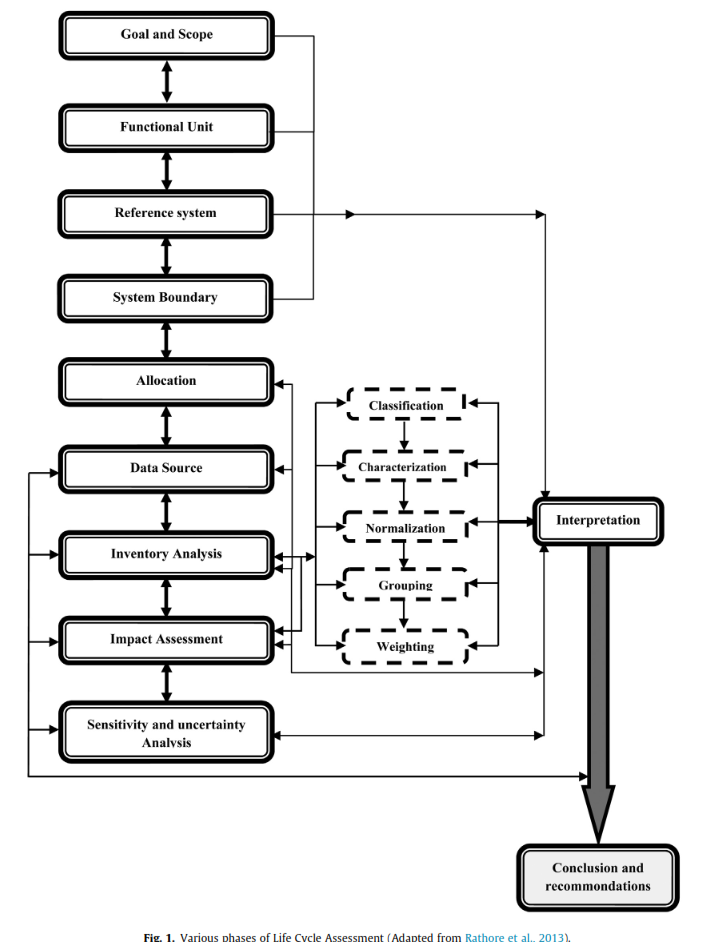
The above LCA discussions suggest that, residue bioenergy have certain environmental superiorities over conventional fuels, if uti- lized in a sustainable way. However, since the same biomass feed- stock can be used through multiple energy conversion routes (heat, electricity, bioethanol), therefore it is important to identify the most beneficial route of conversion. In this regard, Cherubini et al. (2009) reported that (i) production of heat and electricity from biomass has higher GHGs emissions reduction and energy saving benefits than the production of biofuel, (ii) waste/residue biomass shows best environmental performance, since they avoid both the impacts of energy crop production and the emissions from waste management. For enhanced GHGs emissions reduction, Muench (2015) recommended deployment of (i) non-dedicated ligno-cellulosic biomass with thermo-chemical conversion, (ii) dedicated ligno-cellulosic biomass with thermo-chemical conversion and (iii) dedicated ligno-cellulosic biomass with direct combustion. Use of biomass waste/residue has also been suggested as a better emission reduction option than replacing existing croplands or clearing new lands for energy crop plantation
(Fargione et al., 2008; Searchinger et al., 2008; Ho et al., 2014).
Uncertainties in bioenergy LCA
As discussed in the previous section, though the environmental benefits of bioenergy over fossil energy has been realized through LCA studies, however, there are some uncertainties related to LCA applicability (Hellweg and Canals, 2014). The variations may be due to the differences in the type and management of raw materi- als, conversion technologies, end use technologies and the choice of LCA methodologies (consequential/attributional/hybrid LCA) (Cherubini et al., 2009; Muench, 2015; Muench and Guenther,
2013). Lack of general consensus regarding the definition of the system boundary, fossil reference system, optimal functional unit, ideal allocation of environmental impact between products and co- products, modelling carbon cycle of biomass (biogenic/non- biogenic carbon flow) also lead to variations (Muench and Guenther, 2013; Choudhary et al., 2014). In addition, the collection of actual data for LCA study is a challenging task, as these datasets may vary temporally and spatially (Singh et al., 2013). Allocation is a very sensitive issue in LCA which affects the results significantly. The inappropriate allocations could lead to incorrect LCA results. Allocation is a procedure of appropriately allocating the environ- mental burdens of a multi-functional process among its functions or products (Reap et al., 2008). Allocation step is one of the deter- mining steps that tell how much of the environmental burden caused by a multi-functional process should be apportioned to each product or function (Singh and Olsen, 2012). Plevin et al. (2014) cautioned that LCA results should refrain from using unsup- ported claims, such as ‘‘using product X results in a Y% reduction in GHG emission compared to product Z” because such claims are valid only in rare cases.
The limitations of LCA must be taken into account while con- ducting bioenergy LCA. Hellweg and Canals (2014) suggested that it is a duty of the LCA practitioners to explain to the decision mak- ers that LCA is not always a tool to provide a single answer, but it gives a comprehensive understanding of a problem and its possible solutions. Muench (2015) recommended numbers of ways to increase the reliability of LCA results which includes: (i) LCA can be further improved by accounting for heterogeneity among bio- mass systems, (ii) the strong influence of small differences in bio- mass systems must be considered while interpreting the LCA results, (iii) transferability of LCA results between similar systems must always be investigated, (iv) adopting assumptions from other systems should be avoided since it may lead to errors, (v) analysis of GHGs emissions mitigation potential is only a first step in assessing the sustainability of biomass derived electricity and hence future LCA research should include additional environmen- tal, economic and social impact categories. Further, it should also be noted that since a real life situation is modelled in LCA, so, there is a possibility of distortion of the reality in the model outcome (Goedkoop et al., 2013). Hence, developing LCA model with prior knowledge of the system under investigation and careful selection of the system parameters is important to minimize bias between reality and model output and gain a true picture of the environ- mental benefits of bioenergy.
Spatial LCA in bioenergy and environmental planning
Due to the distributed nature of biomass resources, environ- mental impacts of bioenergy may have spatial consequences too, especially with regards to land use and biodiversity. Exclud- ing the impact on biodiversity in LCA studies can significantly limit the applicability of LCA findings (Geyer et al., 2010; Baan et al., 2013). In many bioenergy LCA studies, average country level values are taken as input data. However, parameters such as grain productivity, residue to grain production ratio, surplus resi- due availability, competing uses of residue may vary from region to region. Thus, considering country level average data for local or regional representation may lead to erroneous result. This is par- ticularly true for large scale biomass power plants, where feed- stocks are collected from large geographical areas. Traditional LCAs are unable to recognize the spatial dimension of environ- mental impacts of bioenergy but it can be addressed if the LCA is conducted on a GIS platform (Geyer et al., 2010). Use of Infor- mation Technology supported LCA enables to analyze and visual- ize material flows, processes or products and the calculation of eco-balances on spatial scale (Dresen and Jandewerth, 2012). Applying spatio-temporal models can improve the spatial and temporal depths of LCA analysis (Arodudu et al., 2017). Regional- ized LCA using spatial platform increases the accuracy of assess- ment by accounting site-specific production conditions along with differences in transport and the sensitivity of ecosystems (Hellweg and Canals, 2014). However, limited literature is avail- able on spatial LCA. Therefore, the following discussion is not lim- ited to residue bioenergy but various forms of bioenergy are also covered.
Land use impact on biodiversity is difficult to predict because of the spatial heterogeneity of biodiversity and unavailability of precise impact assessment tool (Geyer et al., 2010). The integrated use of GIS and LCA (spatial LCA) could give important insight into how land use change could impact biodiversity. Geyer et al. (2010) presented a proof-of-concept (Fig. 2) by integrating GIS and LCA together for impact assessment of ethanol production on land use and biodiversity in California. The study found that GIS based inventory modelling of land use allows important refinement in LCA and using GIS, land use can be modelled as a geospatial and nonlinear function of output. Humpenoder et al. (2013) combined GIS based LandSHIFT model with LCA to investi- gate the land use impact on the carbon balance of biofuel in the European Union (EU). The results indicate that land use change has a major impact on the GHGs emissions performance of bio- fuel. In a different study, Geyer et al. (2013) presented a spatial LCA approach of sun-to-wheels energy conversion pathways in the US considering land use impacts, life cycle GHGs emissions and fossil fuel demand for five different sun-to-wheels conversion pathways. Azapagic et al. (2013) developed a decision support system called PUrE which integrates several environmental assessment tools in one platform for sustainability assessment of human activities on the urban environment. The sub- components of the tool includes GIS, LCA, substance flow analysis (SFA), air dispersion modelling (ADM), health impact assessment (HIA) and multi-criteria decision analysis (MCDA). Gasol et al. (2011) conducted a spatial LCA study to investigate decentralized bioenergy potential based on Brassica spp. and Populus spp. The GIS is used to estimate bioenergy production while the LCA is applied to estimate potential CO2 emission reduction from bioen- ergy power plants. Mutel et al. (2012) proposed a GIS combined LCA method for regionalized LCA on spatial platform using Bright- way software which directly includes GIS capabilities in the LCA calculation. Dresen and Jandewerth (2012) combined geo- informatics with LCA to conduct spatial analysis of biogas produc- tion in Germany. Under the HEDGE-BIOMASS project, Ferrarini et al. (2014) proposed a combination of GIS, LCA and SWAT model to investigate landscape level bioenergy production in order to identify how and where bioenergy can be produced sustainably and how to optimize the trade-off between delivery of multiple ecological services and farmers benefit within limited land resources. Integration of GIS into LCA for impact assessment of algal biofuel production from wastewater has also been recently reported by Roostaei and Zhang (2016).
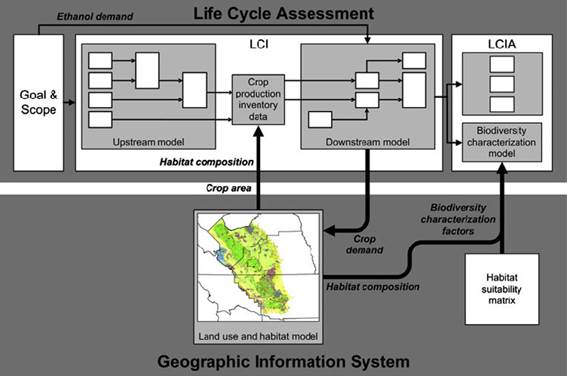
Fig. 2. A procedure to integrate GIS into LCA for the assessment of environmental implications of biofuel production (Geyer et al., 2010). The interface between GIS and LCA are indicated by bold arrows. Here, GIS provides land use land cover and biodiversity habitat data to the LCA framework to identify the bioenergy impact on biodiversity elements.
|