APPLICATION OF GIS IN AGRO-RESIDUE BIOENERGY PLANNING
The whole supply chain of a bioenergy project can be divided into three major spatially interlinked elements: (i) resource assess- ment, (ii) logistic planning, and (iii) power plant design. GIS inter- vention in bioenergy planning is necessary because: (i) diverse varieties of agro-residues are used as energy feedstock, therefore maintaining their spatio-temporal database concerning physico- chemical characteristics, availability and distribution is important. GIS helps in managing such database which later can guide the industries for effective collection of raw material, allocation of the benefits of bioenergy and cost-benefits analysis (Long et al.,2013; Alfonso et al., 2009). Periodic updating of the biomass inven- tories is also necessary to assess future feedstock supply potential; (ii) ensuring sustained feedstock supply is critical for viable com- missioning of a power plant. Prior knowledge of any fluctuation in feedstock supply would allow the user to make necessary arrangement for alternative feedstock supply during lean period of supply and it can be indicated through GIS (Stephen et al., 2010); (iii) consideration of the environmental requirement of resi- due and harvest constraints, economy, technology, competing uses of residue, local socio-political dynamic, land use, logistic facilities, civil and industrial users can also be assessed with GIS (Alfonso et al., 2009; Beccali et al., 2009; Gomez et al., 2010; Jiang et al., 2012).
The uneven geographical distribution of agro-residue demands proper logistic planning for the collection and transfer of residue in a time and cost-efficient manner. The various parties involved in the biomass supply chain that influence the final bioenergy cost include the supplier of biomass, transportation and distribution entities, energy production facility developers and operators, gov- ernment and utility firms and the end users (Mafakheri and Nasiri, 2014). The implementation of a spatial network can help the par- ties involved in bioenergy planning to act in a common framework by sharing costs, logistics, and personnel (Beccali et al., 2009). The rising competition for production areas, raw materials and infras- tructure also demands spatially explicit logistic planning (Fiedler et al., 2007).
Among the logistic parameters, transportation can be cost intensive depending upon the distance and mode of transport (rail- way, roadway, waterway), nature of the feedstock (loose or dense) and the condition of the transport route. Optimization of the trans- portation network can significantly reduce the cost of transport. GIS has the capability to model least-cost transport pathway (Perpina et al., 2009; Ebadian et al., 2011; Jiang et al., 2012; Hiloidhari et al., 2012). For example, Network Analyst extension of ArcGIS software can model least-cost transport pathway for delivering biomass feedstock from source to user location. It allows one to perform multiple network-based spatial functions such as identification of optimal/shortest route, closest facility, service area, origin-destination analysis (Perpina et al., 2009). Further, transporting compacted (baled) biomass reduces cost and CO2 emission, even for short distances by allowing higher amount to be carried (Alfonso et al., 2009).
Another logistic parameter that requires managerial attention is the selection of optimal biomass collection area and power plant site, where multiple factors come into play. Selecting optimal bio- mass collection areas should be in accordance with existing agri- cultural, geographical and infrastructural characteristics of the area encompassing supply and user locations (Beccali et al.,
2009). The site should be easily accessible by transport route, near to utility points, feasible for the optimum planning of power trans- mission lines (Zubaryeva et al., 2012). Further, the plant should be installed in reasonable distance from residential areas, nature reserves to minimize potential negative impacts of plant operation and waste disposal. Beccali et al. (2009) developed a GIS method to assess the techno-economic potential of biomass for energy gener- ation in Sicily through identification of efficient transportation net- work and optimal biomass collection areas. Similarly, Fiedler et al. (2007) designed a GIS logistic model for cost efficient supply of bio- mass feedstock to industrial units by analyzing the profitability of investment in infrastructure and equipment for biomass supply.
GIS based biomass power plant site selection can be done through two methods: (i) suitability analysis (ii) optimally analysis as elaborated by Shi et al. (2008). Suitability analysis allows user to identify the most suitable site for a power plant among many can- didate sites, based on user defined constrain and supportive crite- ria. On the other hand, optimally analysis considers the
relationship between biomass and power plants in order to find the optimal power plant locations at minimum transportation cost. The optimization of biomass power plant location could be done either through location-allocation modelling or supply-area mod- elling. Using the optimally analysis approach, Shi et al. (2008) iden- tified potential sites for biomass power plants in Guangdong Province, China, considering the cost of transportation as a prime determining factor in developing bioenergy plant.
Logistic planning for biomass collection can be further influ- enced by the type of land holding. For example, in India and China, due to small agricultural landholding by the farmer, collecting agro-residue biomass from farmland may be complicated com- pared to the Western countries (Yu et al., 2012). For the same col- lection radius, the collectors have to deal with a larger number of farmers, with organized logistic of contracting, collection and transportation (Yu et al., 2012). In a long supply chain, distributed biomass receiving stations (satellite storage) must be optimized for least cost delivery of biomass as reported by Yu et al. (2012). How- ever, Gomez et al. (2010) reported that, the size of a collection area produces two counteracting effects on the final cost of energy gen- erated. A large biomass collection area results in higher power plant capacity, making the power plant economically reliable, but, it increases the transportation costs of the biomass to the plant. Therefore, the authors (Gomez et al., 2010) have suggested that, in a large scale study with large geographical biomass supply area (e.g. country, province), it is impractical to optimize the size and location of every possible plant. But the better option is to use the same collection area for the whole of the territory and size of this area is to be determined in a way to fulfil the individual plant’s minimum installed capacity (Gomez et al., 2010).
Egbendewe-Mondzozo et al. (2011) proposed a spatial bioeco- nomic model, an integration of biophysical -GIS- economic regio- nal mathematical optimization model to estimate biomass supply from cellulosic crops and crop residues. The GIS part provides the transportation parameters to the bioeconomic model. Overall, the model can predict how biomass supply and environmental conse- quences respond to changes in genetic and biological management as well as market prices and government policy. Similarly, J. Singh et al. (2011) developed a GIS model for agro-residue based decen- tralized biomass power plant design at development block level in Punjab, India, which can be useful to decide optimum power plant locations with minimum storage and handling cost of feedstocks. GIS application has also been found suitable for village level small scale energy planning (Hiloidhari and Baruah, 2011; Kaundinya et al., 2013). Table 1 summarizes selected numbers of recent GIS based bioenergy study.
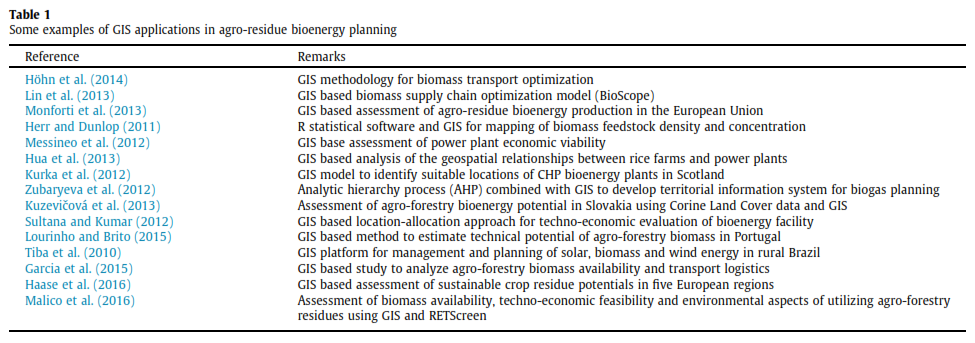
Issues needing attention while using GIS for bioenergy planning
Some issues that could impact the quality of the GIS outputs are discussed below:
(i) Pre and post -GIS analysis field visits to random sample areas are important to ascertain the accuracy of the GIS mapping. Systemic accuracy assessment using standard methods such as computation of error matrix (also called as confusion matrix) can increase the preciseness of the GIS output. Bioenergy planning which involves land use land cover mapping, the minimum mapping accuracy should be
85% (Foody, 2002).
(ii) The usefulness of the GIS output is influenced by the quality of satellite/digital image used for analysis. For example, good quality land use land cover map can be generated using medium resolution image (e.g. LISS-III satellite image of
23.5 meter spatial resolution). However, to map cost- efficient road network for biomass transportation, high res- olution image is necessary (e.g. LISS-IV image of 5.8 meter spatial resolution) to extract all the details of the major and minor roads of a study area.
(iii) Precise image processing in terms of georeferencing, radio- metric calibration, noise removal, image enhancement, post-classification smoothening are other necessary require- ments to achieve higher mapping accuracy.
(iv) The choice of image classification method (manual vs. digi- tal) can also influence the quality of the GIS products.
Therefore, careful consideration of the above points prior to ini- tiating the GIS mapping is essential for precise bioenergy planning.
|