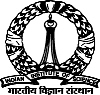 |
Cellular Automata Calibration Model to Capture Urban Growth
|
 |
Uttam Kumar1,4,5, Chiranjit Mukhopadhyay4, T.V. Ramachandra1,2,3*
1Energy & Wetlends Research Group, Center for Ecological Sciences [CES], Indian Institute of Science,
2Center for Sustainable Technologies (astra), Indian Institute of Science,
3Centre for infrastructure, Sustainable Transportation and Urban Planning [CiSTUP]
4Department of Management Studies
5International Institute of Information Technology, Bangalore-560100, India
*Corresponding author:
Energy & Wetlands Research Group,
Centre for Ecological Sciences
Indian Institute of Science,
Bangalore – 560 012, INDIA, E-mail: cestvr@ces.iisc.ernet.in, energy@ces.iisc.ernet.in.
Introduction
Urbanisation is a form of metropolitan growth that is a response to various economic, social, and political forces and to the physical geography of an area. This has resulted in the recent dramatic increase in urban population that is exerting an enormous pressure on the infrastructure services. Many cities are now undergoing a tremendous growth for economic activities with new roads, airports, flyovers, shopping malls, and such other infrastructure developments. This phenomena is very pronounced in India with urban population growing at a rate of around 2.3 percent per annum (Ramachandra and Kumar, 2008; World Urbanization Prospects, 2005). An increased urban growth is irreversible due to population explosion and migration. This dramatic increase in urbanisation has raised the need to understand the dynamics of urban growth process for planning and governance. The impact of piecemeal planning in large cities is often a major concern for stakeholders, such as those involved in researching, modelling, forecasting and policy making, related to planning sustainable urban development (Barredo et al., 2004). It also raises the necessity to understand the dynamics of urban growth process through “growth models” for sustainable distribution of usable resources. Urban growth models with better understanding, prediction and visualisation capabilities have gained relevance in the regional planning with increasing urbanisation in recent times as an emerging research domain. Among these growth models, cellular automata (CA), an artificial intelligence (AI) technique, transportation and land-use planning models based on gravity theory or optimisation, spatial models (Berling-Wolff and Wu, 2004), etc. are being widely used in simulating urban growth processes apart from the conventional mathematical models (Batty and Xie, 1994, Lau and Kam, 2005). CLUE-S (the Conversion of Land Use and its Effects at Small regional extent) simulates land use changes based on empirical analyses of location suitability combined with the dynamic simulation of competition and interactions between the spatial and temporal dynamics of land use systems (Verburg et al. 1999, 2002, 2004, 2010; Veldkamp, 1996). This multi-scale land use change model can predict the impact of biophysical and socio-economic forces that drive land use changes with complex spatial patterns (Verburg and Overmars, 2007; Chen et al., 2010; Xu et al., 2013).
Even though application of CA in urbanisation process modelling have been by and large successful (AlKheder et al., 2006; Batty et al., 1999; Cheng and Masser, 2004; Li et al., 2001; Li et al., 2008), but there are still unresolved issues such as model calibration for reliable prediction (AlKhederet al., 2006, 2007; Li et al., 2001). Since CA models are sensitive to transition rules and their parameter values, calibration aims to find the best transition rule values to reproduce the same urban pattern with reference to historical data. In the literatures, SLEUTH model calibration (Hagerstrand, 1967; Tobler, 1979; von Neumann, 1966; Wolfram, 1994), multi-criteria evaluation (Wu and Webster, 1998), neural networks (Li and Yeh, 2002), genetic algorithms (Alkheder et al., 2006), etc. have been used for calibration. These models involve large input variables with a set of rules which are cumbersome to calibrate. Calibration of CA models requires intensive computation to select the best parameter values for accurate modelling. The present work develops the calibration of a CA model by taking spatial and temporal dynamics of urban growth into account and the technique is demonstrated by capturing the growth pattern of Bangalore city, India with historical remote sensing (RS) and population data.
The paper is organised as follows: The immediate section briefs literature review (focusing on the evolution, development and calibration of CA) and states the objectives of this study, followed by data preparation (classification of RS data and generation of population density maps). Next, CA is introduced with the simulated results and discussion while concluding remarks are presented in the last section.
Citation : U. Kumar, C. Mukhopadhyay, T. V. Ramachandra, 2014. Cellular Automata Calibration Model to Capture Urban Growth. Boletín Geológico y Minero, 125 (3): 285-299 [Best Paper Award, Boletín Geológico y Minero].
|
|
|