Method
A two-step approach was adopted to chart the direction of the City’s development, which includes (i) a normative approach to understand the land use and (ii) a gradient approach of 1km radius to understand the pattern of growth during the past 4 decades. Various stages in the data analysis are:
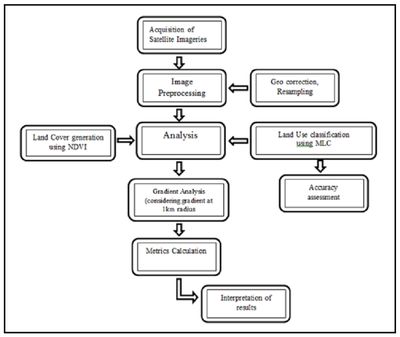
Figure 2: Procedure followed to understand the spatial pattern of landscape change
A. Preprocessing: The remote sensing data obtained were geo-referenced, rectified and cropped pertaining to the study area. The Landsat satellite 1973 images have a spatial resolution of 57.5 m x 57.5 m (nominal resolution) and 1989 - 1999 data of 28.5 m x 28.5 m (nominal resolution) were resampled to uniform 30 m for intra temporal comparisons. Latest data of IRS P6 of spatial resolution 5.6 m was procured from NRSC, Hyderabad (http://www.nrsc.gov.in).
Vegetation Cover Analysis: Normalised Difference Vegetation index (NDVI) was computed to understand the temporal dynamics of the vegetation cover. NDVI value ranges from values -1 to +1, where -0.1 and below indicate soil or barren areas of rock, sand, or urban builtup. NDVI of zero indicates the water cover. Moderate values represent low density vegetation (0.1 to 0.3) and higher values indicate thick canopy vegetation (0.6 to 0.8).
B. Land use analysis: Land use categories listed in Table 2 were classified with the training data (field data) using Gaussian maximum likelihood supervised classier. The analysis included generation of False Colour Composite (bands – green, red and NIR), which helped in identifying heterogeneous area. Polygons were digitized corresponding to the heterogeneous patches covering about 40% of the study region and uniformly distributed over the study region. These training polygons were loaded in pre-calbrated GPS (Global position System). Attribute data (land use types) were collected from the field with the help of GPS corresponding to these polygons. In addition to this, polygons were digitized from Google earth (www.googleearth.com) and Bhuvan (bhuvan.nrsc.gov.in), which were used for classifying latest IRS P6 data. These polygons were overlaid on FCC to supplement the training data for classifying landsat data.
Gaussian maximum likelihood classifier (GMLC) is applied to classify the data using the training data. GMLC uses various classification decisions using probability and cost functions [10] and is proved superior compared to other techniques. Mean and covariance matrix are computed using estimate of maximum likelihood estimator. Estimations of temporal land uses were done through open source GIS (Geographic Information System) - GRASS (Geographic Resource Analysis Support System, http://ces.iisc.ernet.in/grass). 70% of field data were used for classifying the data and the balance 30% were used in validation and accuracy assessment. Thematic layers were generated of classifies data corresponding to four land use categories.
Evaluation of the performance of classifiers [36], [37], [13] is done through accuracy assessment techniques of testing the statistical significance of a difference, comparison of kappa coefficients [8], [47] and proportion of correctly allocated classes [12] through computation of confusion matrix. These are most commonly used to demonstrate the effectiveness of the classifiers [8], [7], [29].
Further each zone was divided into concentric circle of incrementing radii of 1 km (figure 2) from the center of the city for visualising the changes at neighborhood levels. This also helped in identifying the causal factors and the degree of urbanization (in response to the economic, social and political forces) at local levels and visualizing the forms of urban sprawl. The temporal built up density in each circle is monitored through time series analysis.
Table I.A: Land use categories
Land use Class |
Land uses included in the class |
Urban |
This category includes residential area, industrial area, and all paved surfaces and mixed pixels having built up area. |
Water bodies |
Tanks, Lakes, Reservoirs. |
Vegetation |
Forest, Cropland, nurseries. |
Others |
Rocks, quarry pits, open ground at building sites, kaccha roads. |
C. Urban sprawl analysis: Direction-wise Shannon’s entropy (Hn) is computed (equation 1) to understand the extent of growth: compact or divergent [26], [49], [38]. This provides an insight into the development (clumped or disaggregated) with respect to the geographical parameters across ‘n’ concentric regions in the respective zones.

Where Pi is the proportion of the built-up in the ith concentric circle and n is the number of circles/local regions in the particular direction. Shannon’s Entropy values ranges from zero (maximally concentrated) to log n (dispersed growth).
D. Spatial pattern analysis: Landscape metrics provide quantitative description of the composition and configuration of urban landscape. These metrics were computed for each circle, zonewise using classified landuse data at the landscape level with the help of FRAGSTATS [34]. Urban dynamics is characterised by 11 spatial metrics chosen based on complexity, centrality and density criteria. The metrics include the patch area, edge/border, shape, epoch/contagion/ dispersion and are listed in Table II.
Table II: Spatial Landscape Indices
|
Indicators |
Formula |
Range |
Category : Patch area metrics |
1 |
Largest Patch Index (Percentage of landscape) (LPI) |
aij = area (m2) of patch ij
A= total landscape area |
0 ≤ LPI≤100 |
2 |
Number of Urban Patches (NPU) |
NPU = n
NP equals the number of patches in the landscape. |
NPU>0, without limit. |
3 |
Patch density (PD) |
f(sample area) = (Patch Number/Area) * 1000000 |
PD>0 |
4 |
Perimeter-Area Fractal Dimension
(PAFRAC) |
Perimeter-Area Fractal Dimension
aij = area (m2) of patch ij.
pij=perimeter (m) of patch ij.
N= total number of patches in the landscape |
1≤PAFRAC≤2 |
Category : Shape metrics |
5 |
Normalized Landscape Shape Index (NLSI) |
Where si and pi are the area and perimeter of patch i, and N is the total number of patches. |
0≤NLSI<1 |
6 |
Landscape Shape Index (LSI) |
ei = total length of edge (or perimeter) of class i in terms of number of cell surfaces; includes all landscape boundary and background edge segments involving class i.
min ei = minimum total length of edge (or perimeter) of class i in terms of number of cell surfaces. |
LSI>1, Without Limit |
Category: Compactness / contagion / dispersion metrics |
7 |
Clumpiness |
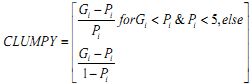
gii =number of like adjacencies between pixels of patch type gik =number of adjacencies between pixels of patch types i and k.
Pi =proportion of the landscape occupied by patch type (class) i. |
-1≤ CLUMPY ≤1. |
8 |
Percentage of Like Adjacencies (PLADJ) |
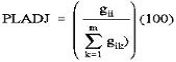
gii = number of like adjacencies (joins) between pixels of patch type
gik = number of adjacencies between pixels of patch types i and k |
0<=PLADJ<=100 |
9 |
Cohesion |
|
0≤cohesion<100 |
10 |
Aggregation index (AI) |
gii =number of like adjacencies between pixels of patch type
Pi= proportion of landscape comprised of patch type. |
1≤AI≤100 |
11 |
Interspersion and Juxtaposition (IJI) |
eik = total length (m) of edge between patch types
E = total length (m) of edge in landscape, excluding background
m = number of patch types (classes) present in the landscape. |
0≤ IJI ≤100 |
|