Data and Method
DATA: Urban dynamics were assessed using temporal remote sensing data for the period 1973 to 2010. The time series spatial data acquired from Landsat Series Multispectral Sensor (57.5 m) and Thematic Mapper (28.5 m), OLI (28.5 m) sensors for the period 1973–2016 were downloaded from the public domain. The Survey of India (SOI) topographic maps of 1:50,000 and 1:250,000 scales were used to generate base layers of city boundary and other details. City maps with ward boundaries were digitized from the BBMP (Bruhat Bangalore Mahanagara Palike) map. Ground control points to register and geo-correct remote sensing data were collected using handheld pre-calibrated GPS (global positioning system), the SOI topographic maps, and online spatial portals. Wetland delineation was performed using field data collected with a handheld GPS. Remote sensing data used for the study are: Landsat MSS data of 1973, Landsat TM of 1992, Landsat ETM+ of 2000 MODIS 7 bands product of 2002 and 2007 downloaded from the online portal, IRS LISS-3 data of 2006 procured from NRSA, Hyderabad. India Google Earth data served in the pre- and post-classification process and validation of the results.
Vegetation cover analysis was done using Indian remote sensing (IRS) satellite Resourcesat 1 (multispectral with spatial resolution of 5.8 m) and Cartosat 1 (panchromatic with spatial resolution 2.7 m). These data were fused using hyperspectral color space resolution merge for better spatial and spectral resolution based on the universal image quality index (UIQI)
METHOD: This includes understanding spatial patterns of urban growth, geovisualization of urban dynamics, vegetation cover analysis, delineation of water bodies, sector-wise analysis of greenhouse gas footprint, changes in land surface temperature, and urban heat island.
Spatial pattern of urban growth in Bangalore:
The remote sensing data obtained were geo-referenced, rectified, and cropped pertaining to the city boundary (study area). Geo-registration of remote sensing data (Landsat data) was done using ground control points collected from the field using precalibrated GPS and also from known points (such as road intersections, etc.) collected from geo-referenced topographic maps published by the Survey of India. The Landsat satellite 1973 images with a spatial resolution of 57.5 m × 57.5 m (nominal resolution) were resampled to 28.5 m comparable to the 1989–2016 data which are 28.5 m × 28.5 m (nominal resolution). Landsat ETM+ bands of 2010 were corrected for the SLC-off by using image enhancement techniques, followed by nearest-neighbor interpolation.
Land-use classification using remote sensing data involved (a) generation of false color composite (FCC), which helped in identifying and locating heterogeneous patches of landscapes; (b) selection of training data from the FCC (the training sites should cover at least 15 percent of the total area and be distributed over the study area); (c) loading of these training data sites as polygon features to a GPS for collection of attribute information for the training data sites from the field; (d) supplementation of the information using Google Earth. Seventy percent of the training data were used for classification and the rest for validation of the classified outputs and accuracy assessment. The classification of the data was completed using GRASS (Geographic Resource Analysis Support System) open source GIS software by considering four prime land-use classes.
Land-use analysis was carried out using supervised pattern classifier Gaussian maximum likelihood algorithm as in Figure 3. This has been proved a superior classifier as it uses various classification decisions using probability and cost functions (Ramachandra, Aithal, and Durgappa 2012). Mean and covariance matrix are computed using estimate of maximum likelihood estimator. Accuracy assessment to evaluate the performance of classifiers was done with the help of field data by testing the statistical significance of a difference, computation of kappa coefficients, and proportion of correctly allocated cases. Recent remote sensing data were classified using the collected training samples. Statistical assessment of classifier performance, based on the performance of spectral classification considering reference pixels, was done which included computation of kappa (𝜅) statistics and overall (producer’s and user’s) accuracies. For earlier time data, training polygon along with attribute details were compiled from the historical published topographic maps, vegetation maps, revenue maps, and so on.
Land-use categories include (a) area under vegetation (parks, botanical gardens, grasslands such as golf courses), (b) built-up (buildings, roads, or any paved surface, (c) water bodies (lakes/tanks, sewage treatment tanks), and (d) others (open areas such as playgrounds, quarry regions, etc.) (see Table 1).
Land use |
Categories included in land use |
Urban |
Residential areas, industrial areas, paved surfaces, mixed pixels with built-up area |
Water |
Tanks, lakes, reservoirs, drainages |
Vegetation |
Forest, plantations |
Other |
Rocks, quarry pits, open ground at building sites, unpaved roads, croplands, nurseries, bare land |
Table 1: Categories included in land use
Modeling and visualization of urban growth
Agent-based modeling was used in the form of fuzzy (to understand the behavior of the agent) as an input, analytical hieratical process (AHP) was used to create the agents’ influence in the region, and CA–Markov to model the land-use transition. Influencing agents were prioritized which include slope, proximity to roads, industry, educational institutions, and bus, railway, and metro stations. Factors such as hospitals and sociocultural buildings have a minimal role in urbanization. All these factors were generalized using a fuzzy analytical process and prioritized using AHP in order to weight the factors based on land use type. The weightages from the AHP and the constraints as Boolean were used to generate transition suitability maps for different land use using multi-criteria evaluation (MCE) technique (Figure 3). The transition suitability (of MCE) along with transition probabilities (from Markov) (were used to calibrate the parameters and simulate the sprawl for the most recent data based in cellular automata using CA–Markov transitions. Validation of the simulated output was carried out with respect to the classified data for recent data. Calibrated parameters were further used to derive the land use for the year 2020
CA–Markov: Markov chains were used to establish the transition probabilities to estimate the area under change based on the transition rules incorporated with the land use. Cellular automata were used to obtain a spatial location and distribution of probable land-use changes based on associated and surrounding pixels. The probability/site suitability map from cellular automata and the transition probabilities were used in convergence in order to simulate the future land-use scenarios.
Fuzzy AHP/CA–Markov: Modeling, simulation, and prediction of the land use dynamics were carried out using the combination of fuzzy logic, analytical hierarchical process (AHP), Markov chains and cellular automata. Factors that have an impact on urbanization include roads, industries, educational institutions, bus stands, railway stations, metro, population, city development plan (CDP), and water bodies were taken into consideration. Fuzzy logic was used to normalize factors such as slope, proximity maps (proximity to road, proximity to industries, proximity to educational institutions, proximity to bus stands, etc.) between 0 to 255, and Boolean algebra was used on constraining factors of development such as water bodies and CDP where the restricted locations for any new change would be zero vice versa. Factors of growth were prioritized/ranked and their effectiveness in change in land use was estimated/mapped using the AHP.
Prioritized weighted factors of growth along with the constraints were combined together through the multi-criteria evaluation process to derive site suitability maps. Site suitability maps provide an insight into how the land use can be managed. The site suitability maps along with the Markov transition probabilities are used in order to simulate the land use for the year 2016 and predict across further years. Both the above section on land use and visualization can be summed up as in Figure 3.
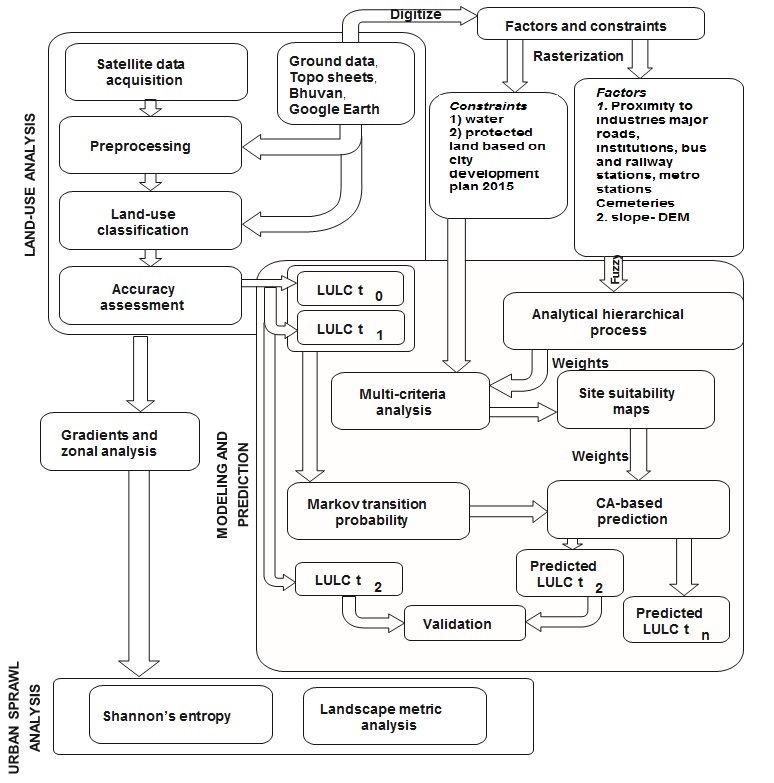
Figure 3: Flowchart illustrating the method of land-use analysis and visualization
Citation : T. V. Ramachandra, Bharath H. Aithal, 2019.Bangalore.The Wiley Blackwell Encyclopedia of Urban and Regional Studies. Edited by Anthony Orum.© 2019 John Wiley & Sons Ltd. Published 2019 by John Wiley & Sons Ltd. DOI: 10.1002/9781118568446.eurs0014
|