Results
Landscape condition analysis
Spatiotemporal quantification of LU transitions in Udupi for
1990-2020 was performed using the Maximum Likelihood
Classification algorithm and accuracy of the classified map was
analyzed by estimating kappa value and overall accuracy. LU map
of Udupi has been shown in Figure 3. The built-up area in the
coastal region increases from 1485 hectares to 33,052.53
hectares, of 8.81% during the three decades. Area under forest
cover i.e., evergreen and deciduous has decreased from 11.74%,
10.47% (1990) to 11.21%, 9.16% (2020) respectively. Scrub forest
increased from 1.85% (1990) to 2.76% (2020). The conversion of
agricultural land to commercial use along the major highways has
increased the built-up area. Horticulture plantations increased
from 36.86% to 43.77% with rubber plantations during three
decades (Table 2).
Figure 3. LU analysis of Udupi from 1990 to 2020
Table 2. Land uses in Udupi District (1990-2020)
Sno |
Land Use Categories
|
1990 |
2018 |
2020 |
|
(Ha) |
(%) |
(Ha) |
(%) |
(Ha) |
(%) |
1 |
Evergreen forest |
42,068.79 |
11.74 |
40,798.62 |
11.38 |
40,178.33 |
11.21 |
2 |
Deciduous forest |
37,466.19 |
10.46 |
29,999.97 |
8.37 |
32,835.18 |
9.16 |
3 |
Horticulture |
1,31,933.07 |
36.82 |
1,56,911.94 |
43.77 |
1,51,911.94 |
42.39 |
4 |
Forest Plantations |
5,090.76 |
1.42 |
7,265.25 |
2.03 |
7,265.25 |
2.03 |
5 |
Croplands |
1,20,544.83 |
33.64 |
90,132.75 |
25.14 |
1,04,305.67 |
29.11 |
6 |
Built up |
1,485.72 |
0.41 |
11,069.64 |
3.09 |
33,052.53 |
9.22 |
7 |
Water |
6,511.77 |
1.82 |
6,309.27 |
1.76 |
8,966.06 |
2.50 |
8 |
Open lands |
6,635.25 |
1.85 |
6,162.57 |
1.73 |
10,136.03 |
2.83 |
9 |
Scrub forest |
6,612.30 |
1.85 |
9,698.67 |
2.73 |
9,876.02 |
2.76 |
Total |
3,58,348.68 |
Figure 4, illustrates that scrub forest increase by 1.37%, and
built-up areas increased by 7.17%. Horticulture plantations
replace agricultural activities during three decades.
Figure 4. Percentage change in LU analysis of Udupi during
(1990-2020)
Fragmentation analyses show loss of intact forest (core),, and
Figure 5 depictsfragmentation fromg 1990 to 2020. Table 3 gives
the spatial extent of various types of fragments (interior
non-forest, patch forest, transitional forest, edge forest,
perforated forest, and interior forest). Area under interior
forest has decreased from 16.77% (1990) to 13.97% (2020) while
non-forest areas has increased from 74.27% (1990) to 78.24%
(2020). The transitional forest has decreased from 1.8% (1990)
to 1.04% (2020), with increased edge forests indicating the
boundary between interior forest and non-forest has increased.
The increase in non-forest areas signifies a major portion of
the forest area has been converted into built-up areas,
croplands, roads, etc. The core forest exists only as protected
areas, sanctuaries, national parks, and sacred groves.
Table 3. Fragmentation Analysis of Udupi District (1990-2020)
Sl.No. |
Components |
1990 |
2018 |
2020 |
Ha |
% |
Ha |
% |
Ha |
% |
1 |
Non-forest |
2,66,144.67 |
74.27 |
2,71,530.63 |
75.77 |
2,80,373.84 |
78.24 |
2 |
Patch |
3573.9 |
1.00 |
2124.18 |
0.59 |
1524.18 |
0.43 |
3 |
Transitional |
6339.15 |
1.77 |
6738.39 |
1.88 |
3738.39 |
1.04 |
4 |
Edge |
2713.59 |
0.76 |
3174.84 |
0.89 |
3174.84 |
0.89 |
5 |
Perforated |
12,963.24 |
3.62 |
12,492.45 |
3.49 |
10,492.45 |
2.93 |
6 |
Interior |
60,102.36 |
16.77 |
55,978.92 |
15.62 |
50,078.92 |
13.97 |
7 |
Water |
6,511.77 |
1.82 |
6,309.27 |
1.76 |
8966.05737 |
2.50 |
Total Area |
3,58,348.68 |
Land Surface Temperature [LST]
The higher temperature can be seen especially in non-forest areas
due to an increase in built-up areas. The temporal LST analysis
shows hilly regions are with moderate surface temperatures,
evident from Table 4. Urban areas show an increase in
temperature (maximum) from 30.71˚C (in 1990) to 37.51 ˚C (in
2018). Due to a reduction in the interior or intact forest
cover, there is an increase in temperature from 29.47 ˚C (in
1990) to 38.94 ˚C (in 2018). Table 4 also illustrates the change
in temperature across land-use categories over the period. The
maximum change in temperature was observed over forest cover
(with the fragmentation of forests) by 6.39 ˚C followed by
non-forest cover by 5.70 ˚C during the past 28 years, comparable
with ground data.
Table 4. Temperature Analysis of Udupi District (1990-2018)
Sno |
Land use categories |
1990 |
2018 |
Change
(˚C) |
Area (%) |
Temp (˚C) |
Area (%) |
Temp
(˚C) |
Min |
Max |
Mean |
Min |
Max |
Mean |
1 |
Forest |
23.95 |
19.38 |
29.47 |
24.43 |
20.31 |
22.68 |
38.94 |
30.81 |
6.39 |
2 |
Non-forest |
73.82 |
19.39 |
31.9 |
25.65 |
74.70 |
23.69 |
38.99 |
31.34 |
5.70 |
3 |
Urban |
0.42 |
22 |
30.71 |
26.36 |
3.18 |
24.38 |
37.51 |
30.95 |
4.59 |
4 |
Water |
1.82 |
20.68 |
28.65 |
24.67 |
1.81 |
23.27 |
37.17 |
30.22 |
5.56 |
Figure 5. Temporal dynamics of LST of Udupi from 1990-2018
Conservation Importance Regions (CIR)
Compilation of attribute data related to ecology, geo-climate,
land, and social aspects has been collected, and aggregated
weight has been computed. Ecology
The ecosystem's health is assessed based on key variables such as
conservation status, diversity, etc. Data is compiled from the
field, review of published literature, and virtual data portals
(such as avibase, ifoundbutterflies, etc.). Conservation
Reserves (CR) have been established under Protected Areas under
the Wildlife Amendment Act of 2002. Conservation Reserves are
essentially the buffer zone between National Parks (NP),
Wildlife Sanctuaries, and reserve forests. Higher weights were
assigned to CR and NP, and the grids with the critically
endangered and endangered species were assigned a value of 10,
vulnerable and near-threatened species are assigned a value of
7, threatened species is assigned 5, common, data deficient,
rare and lower risk were assigned a value of 3 while not
evaluated is assigned a value of 1. Figure 6a-h depicts the
distribution of flora and fauna with conservation status and
weights, which shows most of the species are concentrated across
wildlife sanctuaries in the district.
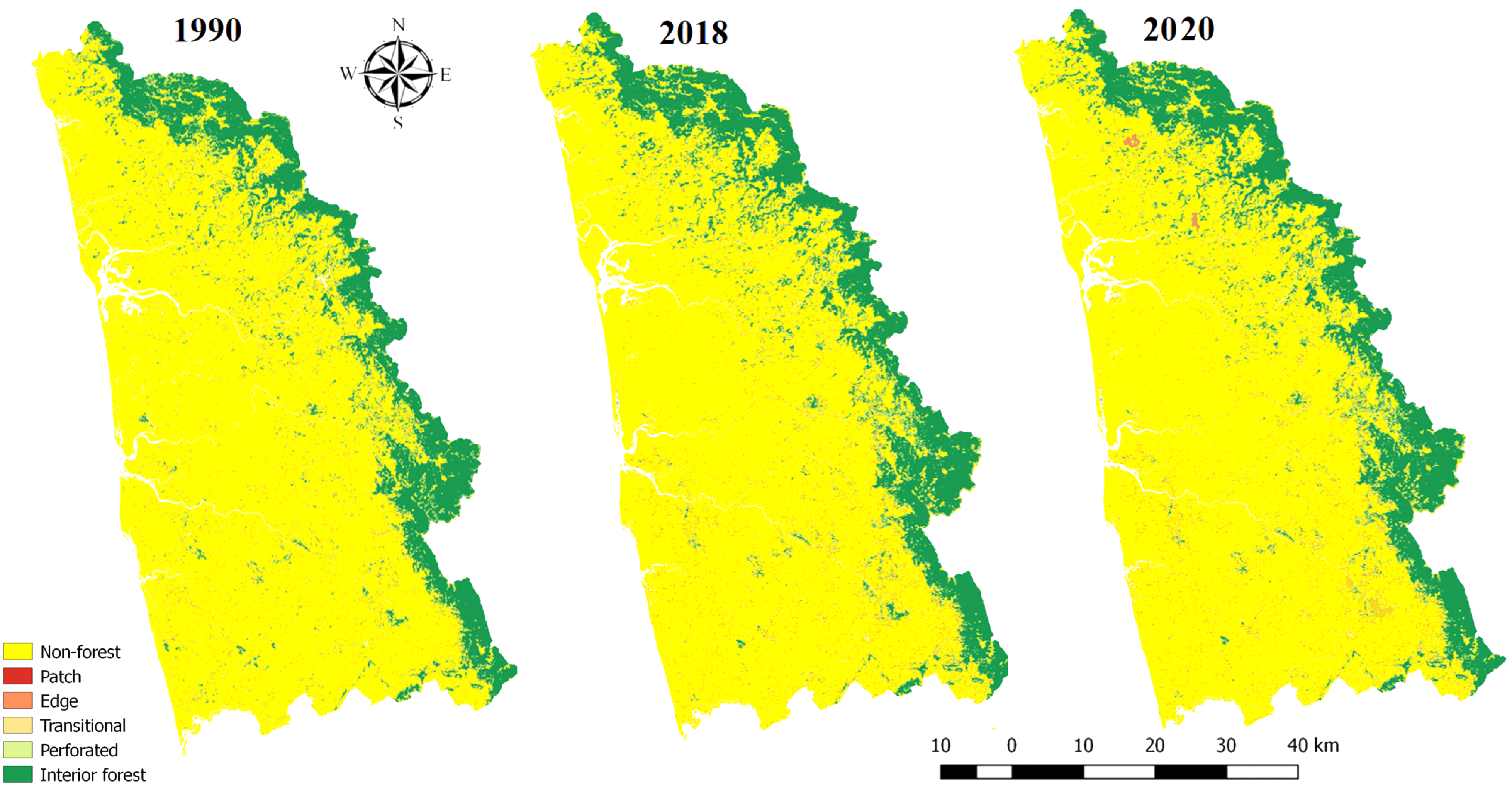
Figure 6. Ecological variables with their weights
The flora of Udupi district has been compiled by reviewing
published literature and data portals. The district is home to
critically endangered species such as Elaeocarpus gaussenii,
Syzygium travancoricum, Utleria salicifolia, Vateria indica,
Vatica chinensis and vulnerable species such as Chloroxylon
swietenia, Cinnamomum sulphuratum, Dalbergia latifolia,
Garcinia indica, Myristica malabarica, Ochreinauclea
missionis, Paracautleya bhatii, Santalum album, Saraca
asoca, Saraca indica. The dominant families are
Fabaceae, Lamiaceae, Dipterocarpaceae, Euphorbiaceae.
Udupi district has rich faunal diversity. The region has
near-threatened amphibian species such as Philautus
beddomii, Ramanella montana, Rana curtipes, Tomoptema
rufescens, and vulnerable species such as Ichthyophis
beddomei, Micrixalus Saxicola, Nyctibatrachus major,
Philautus glandulosus, Rana aurantiaca, Rana leithi.
Mammals species such as Bos gaurus, Cervus unicolor,
Funambulus subineatus, Melursus ursinus, Neophocaena
phocaenoides, Physeter macrocephalus are under near
threatened and species are Hyaena hyaena, Lutra lutra,
Panthera pardus, Ratufa macroura, Sousa chinensis under
vulnerable category of IUCN conservation status. The district
has critically endangered birds such as Fregata andrewsi,
Gyps bengalensis, Gyps indicus, Sarcogyps calvus and
vulnerable category birds such as Chaetornis striata,
Ciconia episcopus, Clanga clanga, Clanga hastata, Columba
elphinstonii, Gallinago nemoricola, Leptoptilos javanicus,
Schoenicola platyurus. The district has vulnerable
category reptiles such as Cnemaspis indica, Cnemaspis
indraneildasi, Cnemaspis jerdonii, Hemidactylus
albofasciatus, Hemidactylus sataraensis, Kaestlea
laterimaculata, Melanophidium bilineatum, Oligodon
brevicauda, Uropeltis phipsonii
Kudermukh National Park covers a minor portion of the study
region and shares its boundary with Dakshina Kannada. The study
region has diverse flora and fauna: 162 species of flowering
plants representing 50 families. Fabaceae had maximum tree
species (23), followed by Rhizophoraceae (17 species), Moraceae
(7 species) – known as the family of figs and keystone species
for plants, etc. Vateria indica is one of the
critically endangered species present in the district while Hopea
ponga, and Syzygium caryophyllatum are the two
endangered species, and Garcinia indica, and Ochreinauclea
missionis are the two vulnerable species that are well
distributed in the district. Someshwara and Mookambika wildlife
sanctuary are known for the variety of birds and wild flowering
species endemic to the region. 816 species of fauna representing
123 families were documented. Malabar Grey Hornbill, Small Green
Barbet, Gotyla, Rock Bush Quail, Grey Jungle fowl, Malabar Wood
shrike, Indian Rufous Babbler, Nilgiri Thrush, Nilgiri Blue
Robin, Day's GlassFish, Gunther's catfish, Tyler’s Leaf Warbler,
etc., are found in the district. Geo-climate
The geo-climatic parameter plays a crucial role in determining
the landscape dynamics of any region. Figure 7a-l depicts the
different geo-climatic parameters in the region and their
weights assigned to the grids. The patterns of altitude, slope,
rainfall helps in determining the forest cover, biodiversity,
etc., of a region. The rainfall pattern shows entire district
receives high rainfall during all seasons. The coastal part
falls under a hot moist sub-humid region can be attributed to a
favorable climatic pattern for healthy vegetation. Grids with
high rainfall and soil types have rich forest cover with high
biodiversity and conservation values. Regions with high
rainfall, elevation, and slope were assigned a value of 10, and
the lowest is given a value of 1. Loamy soil is considered best
for agricultural purposes with rich porosity and humus content,
which is assigned a value of 10, while laterite and mixed red
and black soil are assigned a value of 7, red sandy and medium
black were given 5 and coastal alluvium as 1. Lithology is an
important factor considering landscape structure and patterns.
Charnockites was given the highest value of 10 followed by
peninsular gneiss of 7, closepet granite, and Dharwars were
assigned 5 while alluvium was assigned 1. Agro-ecological zones
play a significant role in determining the climate of a region.
Therefore, hot humid is assigned the highest value of 10 as the
region receives high rainfall around 1500mm or more. Hot moist
sub-humid is assigned 7, hot dry sub-humid is given 5, and hot
moist semi-arid is assigned the lowest value of 3.
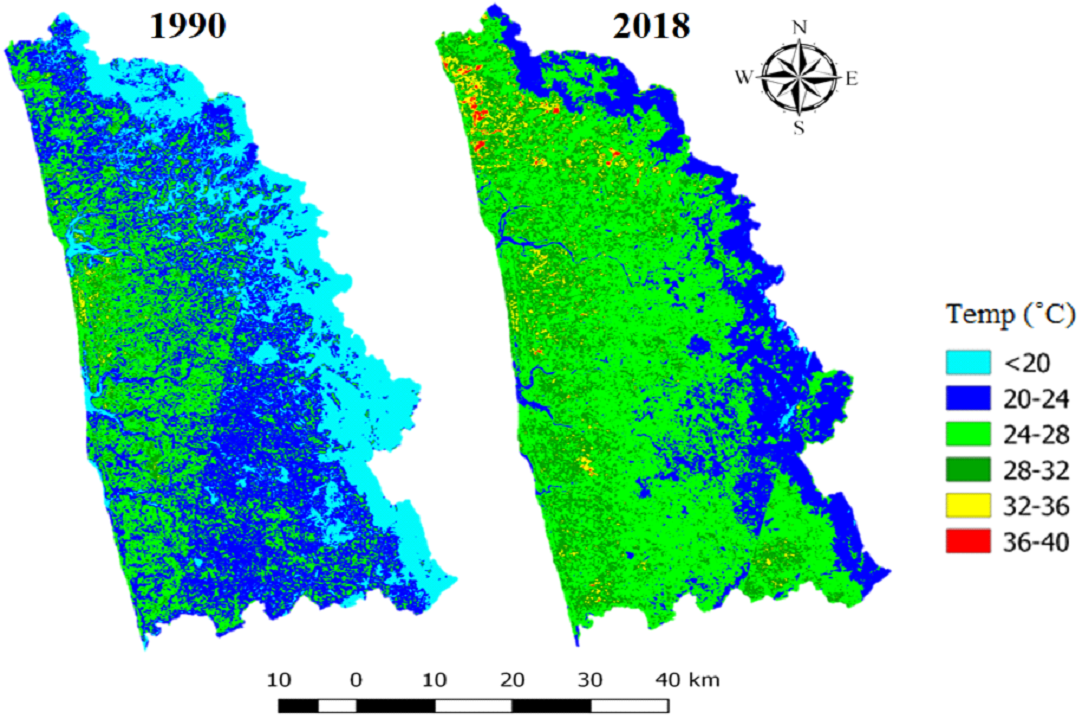
Figure 7. Geo-climatic factors and their weight Land
Grids are prioritized based on the proportion of forest cover.
Forest fragments were computed using standard protocol wherein
interior core forest patches were considered devoid of any edge
effects. Grids having more than 60% forest cover were assigned a
value of 10, and accordingly, values were assigned. Land use
analysis revealed that the region has about 2.98% under
evergreen forest. Most parts are with less than 15% forest
cover. Expansion of agricultural activities and the introduction
of exotic species has led to the destruction of large forest
patches at a temporal scale. The coastal taluks have a forest
cover of less than 15% forest cover. Figure 8 depicts the
weights assigned to the grids based on the extent of forest
cover. Fragmentation analysis revealed that 3.39% of the area is
under core interior forest while 94.25% area is under non-forest
cover.
Figure 8. Land condition factors and weight Social
Population increase often leads to degradation of natural
resources. An increase in population density will lead to loss
of natural resources, species extinction etc. In this study,
population density per sq. km has been considered as one of the
influencing factors in resource use. Therefore, grids with less
population density were given higher weights. Grid-wise, village
population was computed for 2011. The grids with a population
density of less than 50 persons were assigned high weights and
vice-versa. Figure 9 depicts the population density assigned to
the grids with corresponding weights. The hilly regions have
high forest cover with less population density. Forest dwellers
(tribes) of the study regions were mapped, and the grids with a
high tribal population were given higher weight. Forest dwellers
were spatially mapped and were given higher weights as they are
directly or indirectly dependent on forest resources and also
protect the forest.
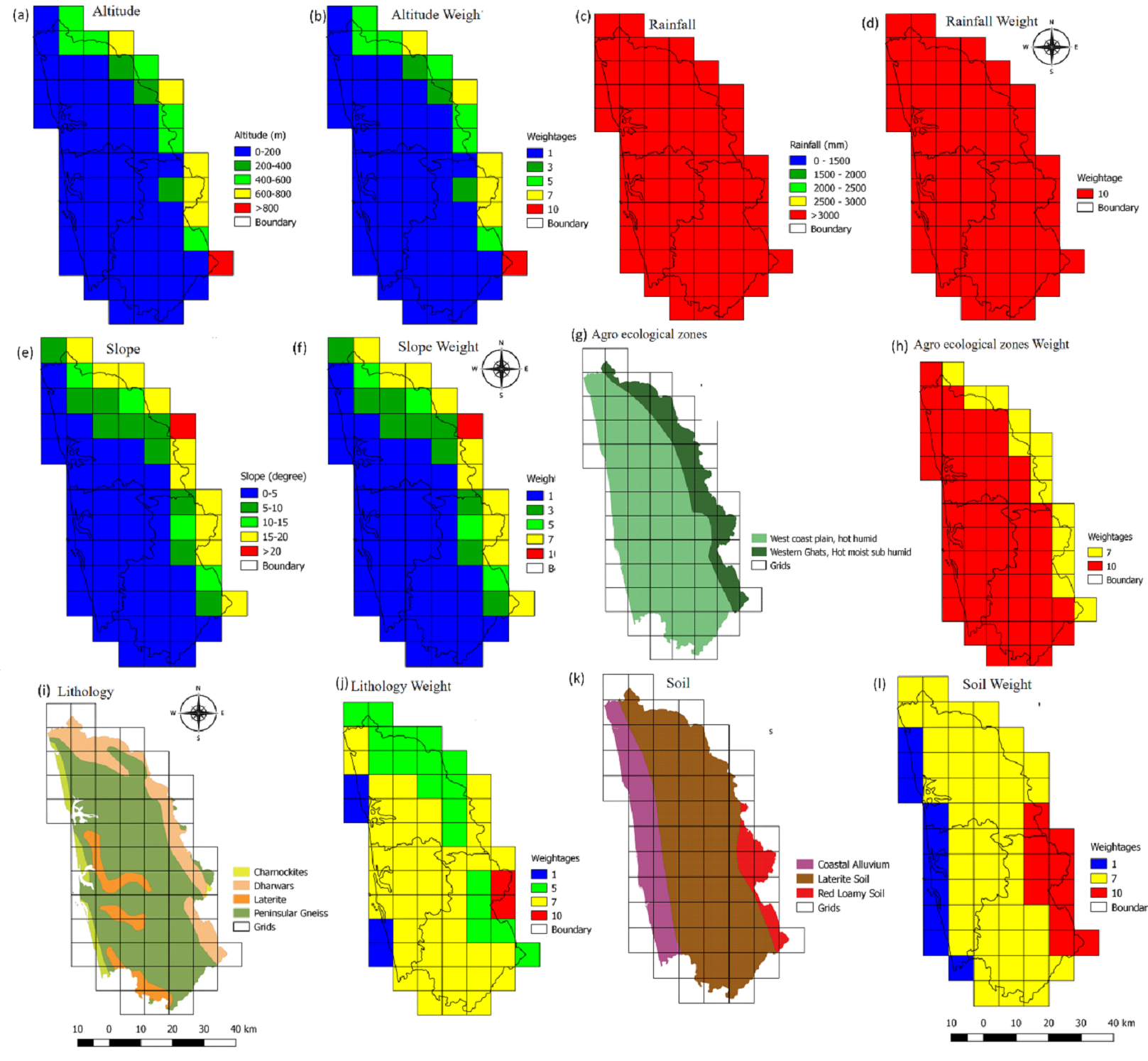
Figure 9. Social factors considered and weight
Based on the relative significance of themes, regions were
prioritized using weightage metric score as CIR 1 (Regions of
highest sensitivity), CIR 2 (Regions of higher sensitivity), CIR
3 (Regions of high sensitivity), and CIR 4 (Regions of moderate
sensitivity). Spatially 15% of the district represents CIR1,
while 31% of the area represents CIR2. 42% of the district
represents CIR 3, and about 12% of the district is in CIR 4.
Figure 10 depicts with taluk and village boundaries. CIR
analysis at the village level shows only 14 villages in CIR 1,
52 villages in CIR 2, 178 villages in CIR 3, and 51 in CIR 4
(Figure 10) .
Figure 10. CIR at taluk and village level- Udupi District
LST in conservation importance
region (ecologically sensitive regions)
The ecological sensitiveness of a region has a direct impact on
temperature. CIR 1has a lower temperature compared to CIR 2 -4.
CIR 1 has the maximum forest cover and rich biodiversity along
with less population, which aided in the low LST. LST trend
shows an increase in temperature (r=0.8) across CIRs (Figure
11).
Fig. 11: Social factors considered and weight
Fig. 12: CIR at taluk and village level- Udupi District
Figure 13. LST across CIR- Udupi
Multivariate analysis is carried out to determine the
relationship between LST and various independent variables
(rainfall, forest cover, interior forest cover, and population
density). In the study, variables considered are rainfall weight
(x1), forest cover (x2), interior forest
cover (x3), and population density weight
(x4) to understand the change of temperature across
the CIR regions in the districts. The probable relationship is
given by
LST = 0 x1- 0.28x2 + 0.16 x3 -
0.28 x4+31.94 (r: 0.851, p <0.05)
|