Materials and Method
Study area
Udupi district (13°04' and 13°59' N latitude and 74°35' and
75°12' E longitude) was formed in the year 1997 with three
taluks i.e., Udupi, Kundapur, and Karkala from the undivided
parent district of Dakshina Kannada. The district is bounded by
Uttara Kannada district in north and Dakshina Kannada district
in southern direction with 3582 square kilometers geographical
area (Figure 1). The district is blessed with abundant rainfall,
fertile soil, and lush vegetation. The slopes of the Western
Ghats are endowed with dense forests containing valuable
species, including timber and fuelwood. The soils of the
district are drained by perennial rivers such as Varahi,
Gangolli, Sitandi, and Swarna, which join the Arabian Sea, known
for its estuarine diversity. Udupi district gets an annual
rainfall of 4000 mm. The climate is marked by heavy rainfall,
high humidity, and sticky weather in the hot seasons. Pristine
beaches, picturesque mountain ranges, temple towns, and rich
culture make it a famous tourist destination. It is well known
for Yakshagana- a fabulous costumed dance-drama form, Kambala-
the buffalo racing sport by farmers, Kori-Katta (Cock Fight),
and Bootha Kola. The district has witnessed largescale
developmental activities post-2000 (Ramachandra and Aithal
2012).
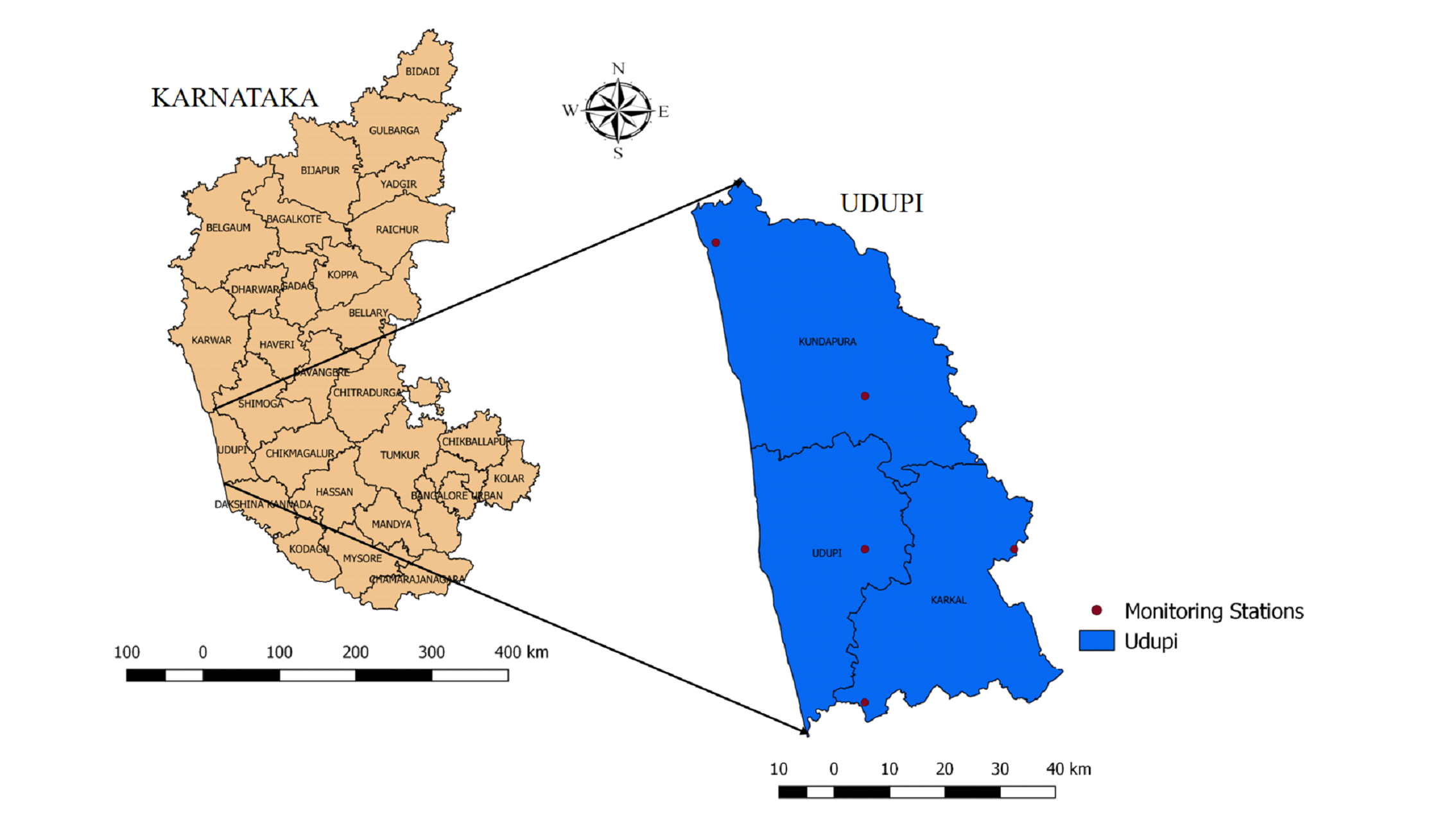
Figure 1. Geographic location-Udupi District
Data
Spatiotemporal cloud-free remote sensing data of Landsat sensors
(1990-2020) has been downloaded from the USGS-Earth explorer
data portal. The Landsat data (all bands including the thermal
band) were geo-registered to a common Universal Transverse
Mercator coordinate system and resampled to 30 m using the
nearest neighbour algorithm. Field data for LU classification
and validation is collected using a pre-calibrated Global
Positioning System (GPS). The data from Google Earth for various
classes like forest, plantation, agriculture, urban, water and
open spaces were also collected and used during analysis and
validation. The temperature data of 2018 was taken from KSNDMC
(Karnataka State Natural Disaster Monitoring Centre), used for
LST validation. Global air temperature data (at 2 m from
ground-based on climate modeling grid of 0.25) is used to
validate LST maps for 1990. A 10 km daily meteorological dataset
of 0.25 deg grid is used to validate LST of 1990. The dataset is
based on the NCEP‐NCAR reanalysis
(https://psl.noaa.gov/data/gridded
/data.ncep.reanalysis.pressure.html#) merged with the University
of East Anglia Climate Research Unit (CRU) monthly gridded
temperature product and the NASA Langley Surface Radiation
Budget (SRB- https://asdc.larc.nasa.gov/ project/SRB) product
and the data are available in ‘NetCDF’ format with one file per
variable per year. Population data of 1901, 2001, and 2011 were
collected from the Census of India
(https://censusindia.gov.in/). Geological data such as soil,
lithology, and agro-ecological zones were obtained from ICAR-
NBSS & LUP (National Bureau of Soil Survey and Land Use
Planning). Elevation data was obtained from USGS EROS Archive -
Shuttle Radar Topography Mission (SRTM) of 30-meter resolution.
Terrain analysis was carried out for obtaining slope. Rainfall
data is obtained from WorldClim- Global Climate Data version-2
(gridded climate data) with a spatial resolution of about 1
km2.
Method
The present study has been carried out in three phases (i) using
remote sensing data to analyse forest ecosystem extent and
conditions (fragmentation), (ii) identify and prioritize
conservation importance regions or ecologically sensitive
regions based on ecology, geo-climatic, land, and social
attributes, (iii) quantifying LST and evaluating the
relationship between CIR and LST.
LU analysis involved,
- Quality parameters: The remote sensing data were chosen to
be devoid of or minimal cloud cover (less than 10 percent)
and pixel quality.
- Image pre-processing and geo-referencing: The data was
rectified radiometric errors and geometric errors. Geometric
rectification is done using ground control points with the
nearest neighborhood technique. The geo-rectified image is
then projected to WGS/UTM 43N (EPSG: 32643).
- LU Classification: Remote sensing data is classified using a
supervised classifier based on the Gaussian Maximum
Likelihood algorithm. In the supervised technique, training
data of representative LU describing the spectral attributes
(Lillesand et al. 2014) is considered. The technique
essentially considers variance and covariance of unknown
pixels (Reddy 2009; Ganasri and Dwarakish 2015; Ramachandra
and Bharath 2019b).
- Validation of LU information: Classified LU information is
validated by accuracy assessment through computation of
error matrix and Kappa statistics. Error matrix compares, on
a category-by-category basis, the relationship between
ground truth data (reference data) and the corresponding
results of the classified data. Kappa coefficient measures
the difference between the actual agreement between the
reference data and classified data and is estimated through
equation 1.
K = (Observed Accuracy-Chance Aggrement) / (1-Chance Aggrement) ..... (1)
Evaluating ecosystem condition:
The condition of the forest ecosystem is assessed through
fragmentation analyses involving both the extent of the forest
and its spatial pattern. Fragmentation of forests measures the
degree to which forested areas are broken into smaller patches
and pierced with non-forest cover. It is estimated through the
computation of Pf and Pff. Pf is
the ratio of the number of pixels that are forested to the total
number of non-forested non-water pixels in the kernel (3 × 3)
and Pff is the proportion of all adjacent (in all
cardinal directions) pixel pairs that include at least one
forest pixel, for which both pixels are forested. Various levels
of fragmentation consist of five components: (i) Interior
forest: It is essentially consisting of thick forest cover, (ii)
Patch forest: Forest area comprising small forested areas
surrounded by non-forested land cover, (iii) Perforated forest:
Forest pixels forming the boundary between an interior forest
and relatively small clearings (perforations) within forest
landscape, (iv) Edge forest: Forest pixels that define the
boundary between interior forest and large non-forested land
cover features and (v) Transitional forest: Areas between edge
type and non-forest types. If higher pixels are non-forest, they
will be tending to non-forest cover with a higher degree of
edge.
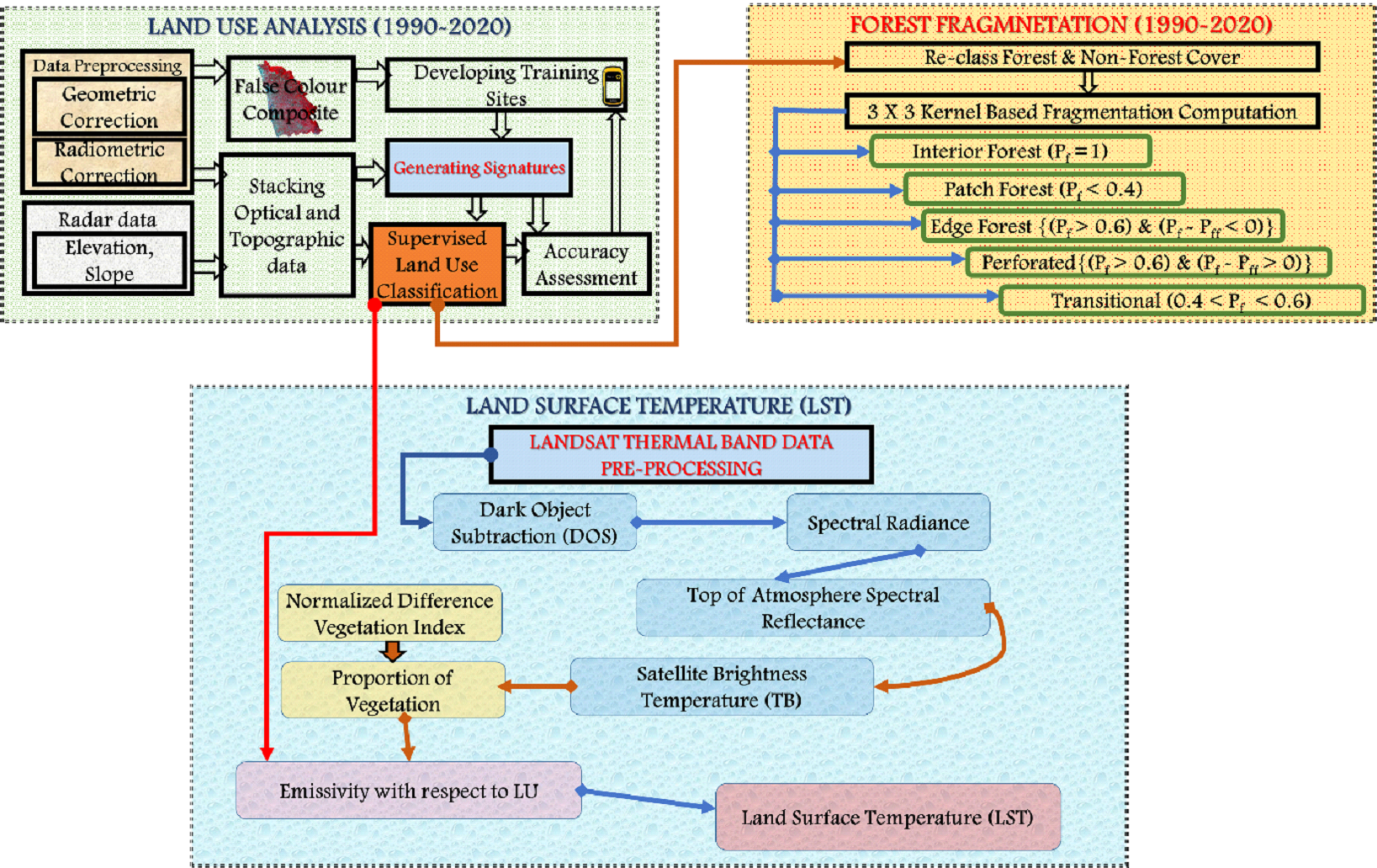
Figure 2. Method adopted for LU, fragmentation, and LST
analysis Land Surface Temperature [LST] estimation
LST is estimated using time series data from top-of-atmosphere
brightness temperatures from the infrared spectral channels of a
constellation of geostationary satellites. Its estimation
depends on the albedo, vegetation cover, and soil moisture
(Bharath et al. 2013; Ibrahim et al. 2016; Sahana et al. 2016).
LST influences the partition of energy between ground and
vegetation and determines the surface air temperature. Retrieval
of LST from Landsat 8 thermal data involves computation of
radiance correction, reflectance correction, converting DN value
to brightness temperature, computation of NDVI, the proportion
of vegetation. Emissivity-corrected LST as follows:
Radiance correctness Lλ = M LQcal+AL ... (2)
Lλis TOA (temperature of atmosphere) spectral radiance (Watts/
(m2 * srad * μm)), is Band specific multiplicative
rescaling factor from the metadata, is Band specific additive
rescaling factor from the metadata, is Quantized and calibrated
standard product pixel values (DN).
Reflectance correctness ρλ′ = Mρ + Aρ 3
ρλ’ is TOA (temperature of the atmosphere) planetary reflectance,
Mρ is Band-specific multiplicative rescaling factor from the
metadata, Aρ is Band-specific additive rescaling factor from the
metadata.
DN value converted to brightness temperature Tb = K2 / ln [(K1/L10)+1] ... (4)
L10 is the spectral radiance of thermal band 10 [Wm−2sr−1μm−1],
is the brightness temperature [Kelvin] and K 1 and K 2 are
constants [Wm−2ster−1μm−1], K 1 = 666.09, K2 = 1282.71.
Calculation of NDVI and Proportion of vegetation (Pv)
using equations 5 and 6, respectively.
NDVI = Band5 - Band4 / Band5 + Band4 .... (5)
Where Band5 is the near-infrared band and band4 is the red
band.
Pv = [NDVI-NDVImin/NDVImax-NDVImin]2 .... (6)
Land surface emissivity is very important for calculating LST as
it is the proportionality factor that scales blackbody radiance
(Planck’s law) to predict emitted radiance, and it is the
efficiency of transmitting thermal energy across the surface
into the atmosphere (Kumari et al. 2018). Emissivity is very
close to 1 for all objects, but to get a precise temperature,
emissivity values for each LC class is separately considered
(Table 1). The land surface emissivity LSE (ε) is calculated as
proposed by Sobrino et al., 2004
ε = 0.004Pv + 0.986 .... (7)
where ε is the emissivity.
Table 1. Table showing LU categories and emissivity values
Land Use types |
Emissivity Values |
Densely urban |
0.946 |
Forest cover |
0.985 |
Non-forest cover |
0.950 |
Water |
0.990 |
Emissivity-corrected LST in degrees Celsius Tb = [Tb/{1+[(λTb/ρ)lnελ]} ... (8)
ρ = h 1.438*10-2 mK Where
σ is the Stefan-Boltzmann constant
(1.38*10−23J/K), h is the Planck's constant
(6.626*10−32Js), and c is the velocity of light
(2.998*108m/s).
Retrieval of LST from Landsat TM is as follows,
Radiance correctness Lλ = (Lmax - Lmin/Qcalmax - Qcalmin)*(Qcal - Qcalmin) + Lmin ... (9)
Lλ is temperature of atmosphere spectral radiance
Qcal is the quantized and calibrated standard product pixel value
(DN)
DN value converted to brightness temperature Tb = [Kb/ln[(K1/Lλ)+1] ... (10)
is the spectral radiance of thermal band 6 [Wm−2sr−1μm−1], is
the brightness temperature [Kelvin] and K 1 a d K 2 are
constants [mW*cm-2* sr-1], K 1 = 1260.56, K2 = 607.76.
Emissivity corrected final LST will be computed using equation
7. Prioritization of Conservation Importance Regions
(CIR)
CIR refers to the areas of high ecological significance value.
These are the regions where anthropogenic activities can cause
alterations in the natural structure of the biological
communities and natural habitats. The steps followed during
identification and prioritization of CIR are detailed in Figure
2 and listed below:
- Creation of grids: The study area is divided into grids of
5’ X 5’ covering approximately 9 X 9 km2
(comparable to grids of the Survey of India topographic maps
of scale 1:50000) for prioritizing CIR at decentralized
levels (panchayat level).
- Integration of data with grid: In this study, four different
attributes being ecology, geo-climatic, land, and social
were selected for the prioritization of CIR. Ecology
consists of flora and fauna present in the region.
Geo-climatic parameters refer to the various geological and
climatic parameters such as rainfall, elevation, slope, LST,
soil, agro-ecological zones, and lithology. Finally, land
essentially consists of forest cover and interior forest
extent and social, composed of tribal and social population
density.
- Weightage metric score: Weightages were assigned to
attributes based on their significance value. The weightage
metric score is estimated using equation 11.
Weightage = ∑i=1 WiVi
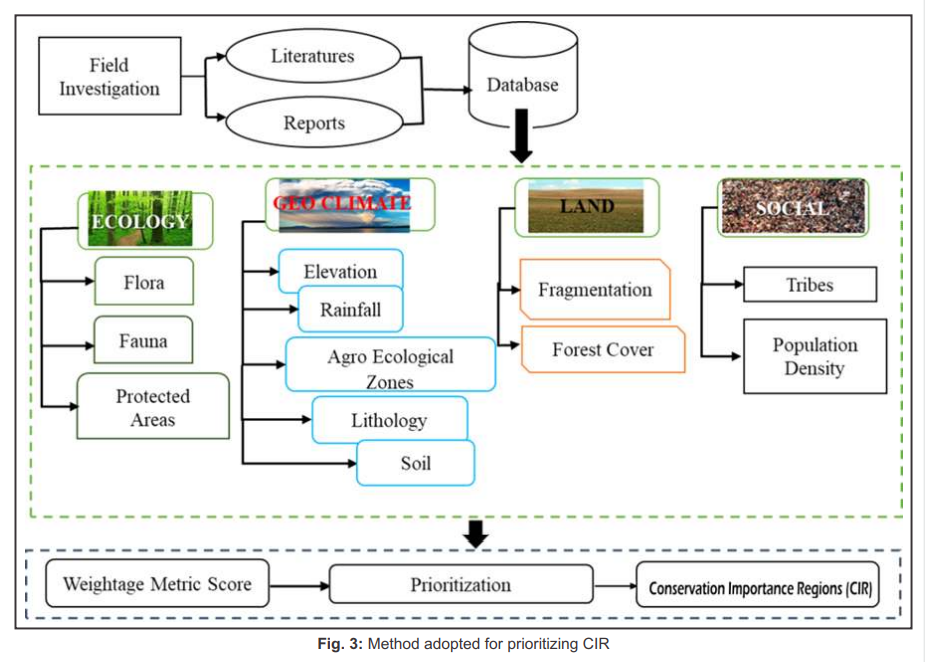
Where n is the number of data sets (variables), is the value
associated with criterion i, and is the weight associated with
that criterion. Based on the weightage, rank is given between 1
to 10 wherein value 10 corresponds to the highest priority for
conservation, 7, 5, and 3 corresponds to high, moderate, and low
level of prioritization, whereas 1 corresponds to least priority
for conservation
- Prioritization of CIR: Weights are aggregated for each grid
and grouped into four groups as CIR 1, CIR 2, CIR 3 and CIR
4 based on the aggregated scores (CIR 1: aggregated scores
> µ+2σ, CIR 2 (for grids within µ+2σ and µ+σ), CIR 3 (for
grids with µ+σ and µ) and CIR 4 (grids with values < µ).
In particular, the weightages are based on an individual
proxy and depends extensively on GIS techniques, which is
the most effective method.
|