Materials and Method
Study Area
Karnataka covers an area of 191,976 km2 (19 million
hectares) with a share of 6% in the national GDP is located in
the southern part of India, sharing borders with Maharashtra and
Goa; Andhra Pradesh and Telangana to the east; Tamil Nadu and
Kerala to the south, while the Arabian Sea forms the western
boundary (Fig. 1). The population of
the state was 611,30,704 inhabitants (as per 2011 census) with a
density of 319 per km2. The state is known for its
diverse culture, scenic beauty, languages, economic, and social
profiles. Karnataka state is divided into four revenue
divisions, 49 subdivisions, 30 districts, 175 taluks, and 745
hoblies/revenue circles for decen- tralized administration. The
Western Ghats, one of the 36 global biodiversity hotspots (https://www.conservation.org)
covers 60% of the state’s forest cover in the western portion
with diverse flora and fauna. The region has diverse forest
cover types such
Fig. 1 Study Area—Karnataka State, India. Source Author
as evergreen, moist as well as dry deciduous, scrub, thorny,
sholas, grasslands, and mangroves in the estuarine areas. The
state harbors 4500 species of flowering plants, 508 species of
birds, 150 varieties of mammals, 156 reptile species, amphibians
of 156 species, 405 fish species, and 330 butterflies. Soils of
the state are fertile by two major river systems (Krishna,
Cauvery) and its tributaries. The state has a protected area
network of five national parks (2431.3 km2) and 21
wildlife sanctuaries (3887.83 km2), covering nearly
16% of forest area. Agriculture and horticulture sectors are the
backbone of the state’s economy. The state is the prime
destination for IT and BT technologies with knowledge,
innovation, research, and development centers. It has a gross
domestic product (GDP) of |15.10 lakh crore (US$220 billion) as
fourth largest in India, growing at a healthy 7% per year with a
per capita GDP of |207,000 (US$3,000).
Method
The protocol adopted to assess the carbon dynamics in Karnataka
is presented in Fig. 2. The research
involved (i) assessment of land-use dynamics through spatial
data acquired using spaceborne sensors at regular intervals;
(ii) field data collection to classify remote sensing data,
(iii) quantification of AGB through field measurements of girth
and height and sampling of the locations through transect based
quadrat;
(iv) quantification of carbon across various forest types and
soil; (v) data mining pertaining to carbon emissions,
sequestrations in forests and soils through published
literature; (vi) visualization of likely changes in carbon
dynamics (a) with the current rate of deforestation and
degradation; (b) interventions with the afforestation; (c)
implementation of the proposed development projects. This was
implemented in three phases. Phase 1 focused on the land-use
analyses, Phase 2 estimates the carbon sinks as well as its
variation over time; quantified the emissions across each sector
followed by carbon budgeting, and likely changes in carbon
dynamics are predicted in Phase 3.
Land-use dynamics—Spatial patterns of land-use dynamics
assessment using temporal remote sensing data: The remote
sensing data of Landsat series for 1985, 2005, 2019 (downloaded
from the public domain https://landsat.org)
were analyzed through efficient supervised classifier based on
GMLC (Gaussian Maximum Like- lihood Classifier) algorithm using
free and opensource GRASS GIS (Geographical Analysis Support
System—https://wgbis.ces.iisc.ernet.in/grass/).
The field investi- gation has been carried out for collecting
training data, which was used to classify the remote sensing
data of 2019 coinciding with the field data collection period.
The earlier time remote sensing data were classified using
collateral data compiled from various sources such as Karnataka
Forest Department reports (https://aranya.gov.in),
vegetation map of South
India of 1:250,000, the French Institute of India (https://
www.ifpindia.org). The process of remote sensing data
classification involved (i) preparation of false-color
composite (FCC) using five bands (R, G, and NIR) of LANDSAT
satellite data, which assisted in the selection of training
sites through the
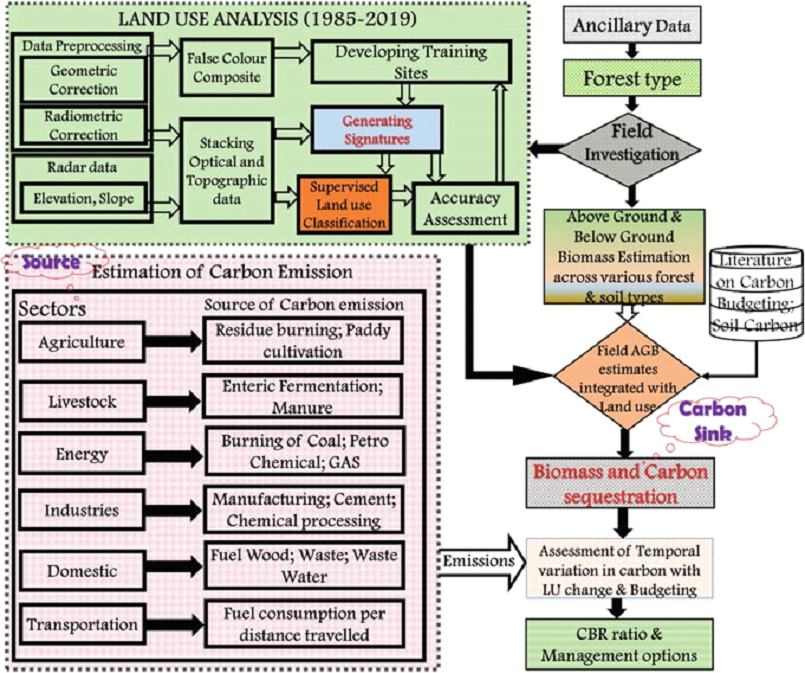
Fig. 2 Method adopted for
carbon budgeting for Karnataka. Source Author
identification of heterogeneous patches corresponding to diverse
landscape elements,
(ii) attribute data collected in the field corresponding to these
training polygons using precalibrated GPS (Global positioning
system) and virtual data (Google Earth— https://earth.google.com,
Bhuvan https://bhuvan.nrsc.gov.in),
(iii) classification of RS data for eight different land-use
categories through GMLC algorithm using training data, and (iv)
accuracy assessment of classified remote sensing informa- tion
was done through error matrix (contingency matrix) and
KAstatistics (Kappa). The training data
compiled from field (60%) have been used for classification,
while the balance is used for accuracy assessment and validation
[28].
Estimation
of Spatiotemporal Carbon Sequestration Potential
The carbon sequestration potential of forest ecosystems,
plantations, and agricul- ture areas was assessed based on (i)
field estimations carried out in the forests across Karnataka
state using transect cum quadrat based sampling techniques and
(ii)
published literature based on the rigorous distinctive biomass
experiments. The study region (Karnataka State) was divided into
2597 grids of 51 51 (or 9 km 9 km) grids
corresponding to 51 51 grids of 1:50,000
topographic maps of the Survey of India. Select grids
corresponding to agroclimatic zones were chosen for biomass and
carbon estimation through field investigations. The basal area,
height, vegetation type (evergreen, deciduous, semievergreen,
moist deciduous, scrub forests), diver- sity, biomass, carbon,
etc., were estimated aiding field data. The comprehensive field
evaluations were done across various forest cover types with
about 424 transects in Uttara Kannada, Dharwad, Shimoga, Udupi,
Chikmagalur, Dakshina Kannada, and Kodagu districts. The number
of quadrats per transects varied between 3 and 5 depending on
the occurrence of species in the sampling locality. The biomass
was estimated using GBH or DBH (girth/diameter at breast height)
for the trees >30 cm. The transect data and standard
literature data were used for biomass quantifica- tion. The
biomass, annual increment in biomass of various forest types,
sequestered carbon, productivity have been computed using field
data integrating with the infor- mation compiled from
literature, which are listed in Tables 1 and 2.
The probable relationship of biomass with the vegetation cover
has been evaluated through multi- variate regression analysis
across coastal, Sahyadri, plain regions of Karnataka. The carbon
for above ground vegetation is computed as 50% of AGB value. The
carbon is deposited in the soil as soil organic matter in both
organic (SOC) and inorganic forms. SOC is calculated based on
the field estimations in top 30 cm soil for different forests
(Table 3) and mean soil carbon
reported in the literature [31, 55, 40].
Quantification
of Carbon Emission from Various Sectors
Data pertaining to emission for sectors such as agriculture,
livestock, industry, energy, transportation, etc., were compiled
from published literature. Agriculture
Agricultural residue burning is practiced in some taluks across
Karnataka. Emissions due to crop residue burning were computed
as per the guidelines of IPCC and liter- ature [11, 61] based on the area of crop
grown to the standard crop residue ratio. The total emission is
estimated by summing of CO2; methane (CH4)
and Carbon monoxide (CO) (its equivalent CO2) values
[17, 33].
The emission from agriculture residue (ARe) burning
is estimated as,
ARe = Σ3i=1 Σ[Cropresidueratio
× emissioncoefficient]
Table 1 Forest type-wise
quantification of biomass and sequestered carbon
Index |
Forest type |
Equation |
Quantification |
Biomass (T/Ha) |
Evergreen |
(Forestcover) × 485.67
|
Above ground biomass content |
Deciduous |
(Forestcover) × 258.12
|
Scrub |
(Forestcover) × 74.25
|
Plantations |
(Extent) × 45.25 |
Carbon stored (T/Ha) |
All |
(Estimatedbiomass) × 0.5
|
Sequestered carbon |
Annual Increment in Biomass (T/Ha)
|
Evergreen |
(Forestcover) × 10.48
|
Incremental growth in biomass
[8, 10, 35, 40, 46, 48] |
Deciduous |
(Forestcover) × 13.82
|
Scrub |
(Forestcover) × 5.4
|
Plantations |
(Extent) × 1.4 |
Annual increment in Carbon (T/Ha) |
All |
(AnnualIncrementinBiomass) ×
0.5 |
Incremental growth in carbon storage |
Net annual Biomass productivity
(T/Ha) |
Evergreen |
(Forestcover) × 3.6
|
Used to compute the annual availability
of woody biomass in the region |
Deciduous |
(Forestcover) × 3.9
|
Scrub |
(Forestcover) × 0.5
|
Carbon sequestration of soil (T/Ha)
|
Evergreen |
(Forestcover) × 132.8
|
Carbon stored in soil [7, 38, 55]
|
Deciduous |
(Forestcover) × 58 |
Scrub |
(Forestcover) × 44 |
Agriculture |
(Extent) × 2.43 |
Plantations |
(Extent) × 55 |
Annual Increment of soil carbon (T/Ha) |
All |
(Cover) × 2.5 |
Annual increment of carbon stored in the soil
|
Table 2 Above ground
biomass for different forest types and plantations
Sno |
Forest cover type |
Standing Biomass (T/ha) |
Source |
1 |
Dense Evergreen to Semi evergreen |
486–834 |
Field-based transect cum quadrat
method;
[20, 37, 38, 40, 43, 46–48, 53] |
2 |
Low evergreen |
226 |
3 |
Dense Deciduous |
258 |
4 |
Degraded Deciduous |
130 |
5 |
Savanna Woodlands |
75–90 |
6 |
Thorn degraded |
40 |
7 |
Littoral and swamp |
215 |
8 |
Plantations |
45–126 |
Table 3 Soil carbon
storage in different forest types and agriculture filed
Sno |
Forest cover type free and opensource |
Mean SOC in top 30 cm (t/ha) |
Source |
1 |
Tropical Wet Evergreen Forest |
132.8 |
[20, 22, 40,
54, 55, 62] |
2 |
Tropical Semi Evergreen Forest |
171.75 |
3 |
Tropical Moist Deciduous Forest |
57.14 |
4 |
Littoral and Swampy Forest |
34.9 |
5 |
Tropical Dry Deciduous Forest |
58 |
6 |
Tropical Thorn Forest |
44 |
7 |
Tropical Dry Evergreen Forest |
33 |
8 |
Agriculture Fields |
4 |
9 |
Plantations |
55” |
Livestock
Livestock plays an important role in the agroecosystem, apart
from the critical energy input to the croplands, also provides
economic support to the farmers in terms of milk, manure, soil
nutrient enrichment, etc. Livestock also produces CH4
emissions from enteric fermentation and CH4 and
N2O (nitrous oxide) emissions are from manure
management systems. The agriculture sector accounts for
approximately 20 and 35% of global GHG emissions [11]. The grid-wise livestock
density has been estimated and associated emission was
quantified under enteric fermentation as well as manure
management [9, 21, 60]. Livestock population
(Census 2012) data were obtained from the State Veterinary
Department, Government of Karnataka, and respective emission
factors are listed in Table 4. CH4
emissions (kg CH4/animal/year) due to the enteric
fermentation are computed as,
Table 4 Emission factors
associated with livestock
Livestock variety |
Emission factor(Kg/Head/Yr) |
Enteric fermentation |
Manure management |
Cattle |
Indigenous |
34.05 |
3 |
Crossbred |
29.42 |
3.46 |
Buffalo |
54.28 |
3.36 |
Sheep |
3.67 |
0.16 |
Goat |
4.99 |
0.17 |
Others |
8.64 |
4 |
CH4EntericFermentation = ΣI(EFI ×
NI)/106 (2)
where, EFI is an emission factor for the individual
livestock category, NI is the number of animals of
livestock for category I. The emission from manure depends on
volatile solids or ruminants, their productivity, and manure
handling system [51]. Methane
emission due to manure management is estimated as,
CH4Manuremanagement = ΣI(EFI ×
NI)/106 (3)
where EFI is an emission factor for each livestock
category; NI is the number of livestock for category
I in the region. Further, CO2 equivalent values have
been estimated across the grids for the fermentation and manure
emissions.
Paddy cultivation is another major activity across the globe,
contributing for 20% methane emission [64]. Paddy is grown in all
taluks of Karnataka state and emission from paddy (Oryza
sativa) is estimated across the grids as,
CH4Paddy = [EF × T × A] (4)
where EF is the daily emission factor (kg CH4/Ha/Day),
T is the cultivation period, A is the harvested area (in two
seasons-Kharif; Rabi). Domestic
The fuelwood consumption is causing deforestation and an increase
in C02 emis- sion. The Per Capita Fuel Consumption (PCFC) was
analyzed to account fuelwood consumption pattern across the
agroclimatic zones of state and determined the carbon emissions
(EFC) as,
EFWC = [NH × PC FC × EF ] (4)
where EFWC is carbon emission from fuelwood consumption in rural
households, PCFC is per capita fuel consumption (which was
computed as ration of fuelwood consumed in kgs/day and number of
adults in a household), EF is emission factor.
The waste generated per household level is also contributing to
the CH4 emissions due to the disorganized management
of waste across the state. The waste generated across individual
households of Karnataka at the grid level has been estimated
consid- ering 0.35 g per person per day [45].
The average of four people per household was considered for a
total of 2,281,419 households. The emission from waste per year
is calculated as,
EWC = [0.35 × NH × 365] (5)
where EWC is carbon emission from waste generated, NH is the
number of households.
Nitrous oxide (N2O) emissions can occur as both direct
and indirect emissions, apart from CH4 through
domestic wastewater, which has significant carbon loading [16]. The emission from
wastewater generated from the individual households is estimated
by considering average water consumption per person per day as
135 L [39, 45, 59]. Industries
Karnataka state is endowed with rich mineral resources as well as
a large pool of human resources. The state has public sector
units and also gives impetus simul- taneously to private sector
growth, which prompted to establish many industries. The state
has major manufacturing industries due to progressive industrial
policies. The good Institutional networks such as Search Results
Karnataka Industrial Area Development Board-KIADB (en.kiadb.in),
Karnataka State Small Industries Devel- opment Corporation
Ltd-KSSIDC (kssidc.co.in), Karnataka State Small Industries
Development Corporation Ltd-KSSIMC, Technical Consultancy
Services Organi- sation of Karnataka-TECSOK (tecsok.com),
Federation of Karnataka Chambers of Commerce and Industry-FKCCI
(fkcci.org), and Industries and Commerce Depart- ment
(kum.karnatka.gov.in) were set up to provide various assistance
for industrial development in the state. The major manufacturing
industries such as cement, steel, iron ore processing,
petrochemical, sugar, paper, and paper board, etc., were consid-
ered [12] and associated emissions
[51] were estimated based on the
standard protocol (Annexure-I). Energy
The energy sector is considered to account emissions from thermal
(burning of coal) and diesel power generation. The state has an
installed power generation capacity of 28,789.99 MW of which,
central utilities contribute 4123 MW, private utilities
contribute 13,259.71 MW and 11,407.28 MW under state utilities.
The thermal power contributes 9,560.82 MW, 698.00 MW by nuclear,
and 8,431.34 MW by renew- able energy sources for the total
installed power generation capacity. The various thermal and
diesel power generating units were mapped across the state and
emissions associated were estimated (Annexure-II).
Transportation
Karnataka stands fifth as per registered motorized vehicles and
contributing 7% of registered vehicles of India. Bengaluru has a
large quantum of vehicles after Delhi with higher vehicle
registrations. The quantum of registered vehicles in the
state
has been gradually increasing at an average growth rate of 10%
per annum and the decadal growth rate of vehicles at 138%. The
two-wheelers account for 70% of the registered vehicles across
the six divisions (Table 5). The
emission from each type of vehicle was evaluated by computing
annual average distance traveled (AADT) [15,
39, 44].
The total emission from the transportation sector has been
quantified as,
Et = ΣVi × AADT i
× EFi, jkm (6)
where, Et is the total emission from the
transportation sector, Vi Number of vehicles per type
i, AADTi is the annual average distance traveled per
different vehicle types and EFi,j,km is the vehicle
type (i) emission of factors (j), per driven kilometer (Table 6).
Carbon Ratio (CR)
CR was computed as a ratio of total carbon uptake (from AGB, SOC)
to the total emissions across all sectors, which will provide
the carbon status across the grids in Karnataka. CR values of
“0” and close to 0 represent the regions of higher emission and
value greater than 1 represent carbon sequestration is higher in
that grids.
CR = Σ(Carbon Sequestration)/Σ(Carbon Emission)
|