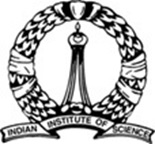 |
Modeling urban dynamics along two major industrial corridors in India |
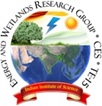 |
Results and Discussion
Industrial corridors have been planned and promoted by the Government of India as effective instruments for achieving accelerated industrial growth by integrating industry and infrastructure with the available local resources based on cluster-based developmental path, to attain accelerated development and regional industry agglomeration. In this regard, the academia, government and industry collaboration would aid in the planned urbanization with innovations.
NDVI [42] aided as prelude knowledge for LU analysis and field data collection. Details of land cover analysis are summarized in Table 3 and is presented in Fig. 3. Vege-tation cover has declined during the last three decades from 40.55 to 23.99% in the Mumbai–Pune (MPI) corridor. Chennai–Bangalore–Mangalore (CBM) recorded a decrease from 44.08 to 37.29%.
Spatial patterns of land uses were analyzed using remote sensing data through supervised classifier and are depicted in Fig. 4, which highlights that built-up area has increased by about four times during the past three decades in both corridors. Built-up area or paved surface has increased from 3.66% (in 1997) to 19.81% (in 2015) along MPI corridor, with the reduction in vegetation from 41.27 to 24.64% (Table 4). MPI corridor is located in one of the global biodiversity hotspots of India, which also witnessed severe erosion in vegetation cover with the opening up of the corridor for transportation in 2000. Land use analysis in
MPI corridor shows that Mumbai, Navi-Mumbai, and Pune city regions are witnessing intense urbanization process with both outward and inward growth such as ribbon development, sprawling and infilling. Lonavala region located between these major cities has witnessed sprawl (from both cities) to form intense development.
Similarly, along the CBMI corridor, built-up area has increased from 2.27% (in 1997) to 8.54% (2014). Bangalore and Chennai area urbanizing at higher stride compared to other cities/towns such as Mangalore, Vellore, Hosur, Kancheepuram, Kunigal, Hassan, etc. Water bodies in and around Bangalore and Chennai are also undergoing stress with the unplanned urbanization.
Spatial patterns of landscape dynamics are assessed in each segment using landscape metrics for both the study regions. MPI corridor regions consists of five segments (Fig. 5a), while CBMI corridor region consists of 25 seg-ments (Fig. 5b). Modelling was performed considering the neighborhood, constraints in the neighborhood and other factors.
Land use modeling was carried out using CA-Markov integrated with Fuzzy ABM framework. The behavior of agents was captured through Fuzzy (similar to human thinking) with rule-bound and context-specific formulation of rules and the urbanisation data of the existing Mumbai Pune express highway (operational since 2002), that improved the connectivity while reducing fright time of goods and services. This has led to an escalation of industrial activities with job opportunities along the corri-dor closer to Mumbai and Pune cities. Population dynamics along the corridor between 2001 and 2011 indicated ram-pant growth at outskirts of Mumbai and Pune indicting high development in these regions. This highlights the role of the corridor in urbanisation with agents such as population followed by other agents of change (road networks, bus stops/railway stations, Schools and Other educational
institutes, industries, Places Social-Cultural importance, distance to CBD, connectivity through airports etc.). This assists AHP in the numerical computation to achieve influential pattern around each agents and objective behavioral influences in each pattern. Fuzzy-AHP is used to include the effects of the agent-based influence on the land use. Markov chains are used to understand the change probability of each land use between t1 and t2. Markov transition probability matrix for Mumbai–Pune and Chennai Mangalore area as shown on Tables 5 and 6 respectively. CA-Markov is then used do the space–time allocation with probability changes using defined rules and neighborhood criteria of each cell. The integration of agents to CA Markov is then used to simulate the likely changes in the two industrial corridors.
During 2009–2015, other land use category has a probability of 28% to change to built-up and vegetation has a probability of 30% to change to other land use category (Table 5) in MPI corridor.
Similarly, in CBMI corridor, during 2009 to 2014, other land use category has a probability of 19.2% to change to built-up whereas and vegetation has a probability of 33% to change to other land use category (Table 6).
Validation of the simulated land uses reveals of higher accuracies for CBMI (overall accuracy of 92.5%, kappa of 0.93) and for MPI (overall accuracy of 89.63%, kappa of
0.87). Simulated results of land uses are listed in Table 7 and are presented in Fig. 6a, b for MPI and CBMI respectively. As the accuracy of simulation was good, land uses for 2021 and 2027 are predicted with the constant time scale for MPI corridor, which are presented in Fig. 7 and details of the likely land uses are listed in Table 8. Urban growth along the corridor is predicted to increase from 19.81% (in 2015) to 47.12% (by 2027). Pune, Mumbai and
Navi Mumbai would be saturated by 2021 and outward concentrated growth would continue. High urban growths can be observed in close proximity to industries along the corridor. Vegetation is expected to reduce from 24.6% (in 2015) to 11.1% (in 2027). Ambivali, Panvel, Chinchwali, Khopli, Dehu showed rampant growth which can be attributed to the connectivity and proximity of the corridor.
Similarly, land uses for 2019, 2024 and 2029 are pre-dicted of CBMI corridor, which are presented in Fig. 8 and likely land uses statistics are listed in Table 9. Urban growth in CBMI region is predicted to increase from 8.54% (in 2014) to 35.4% (by 2029). Bangalore and Chennai cities would be saturated by 2024 and would witness concentrated growth in peri-urban regions. High urban growths can be observed in close proximity to industries and CBDs along the corridor namely Mangalore, Hassan, Kunigal, Vellore, Kancheepuram, Hosur. Vegetation is expected to reduce from 40.8% (in 2014) to 10.6% (in 2027).
|
|
Citation :T.V. Ramachandra, Jeffery M. Sellers, H. A. Bharath, S. Vinay, 2018. Modelling urban dynamics along two major industrial corridors in India. Spatial Information ResearchISSN 2366-3286Spat. Inf. Res.DOI 10.1007/s41324-018-0217-8
|