Materials and Methods
Study Area
Bengaluru (also referred as Bangalore), the capital of Karnataka State, India, is the
cosmopolitan city located at 949 m asl with the spatial extent of 741 sq. km. Bangalore has
grown spatially more than ten times (741 sq. km) since 1949 (69 sq. km.). Due to rapid urban
growth, Bangalore has been experiencing changes in the temperature and is becoming an urban heat
island (Ramachandra and Kumar, 2010). The unrealistic unplanned growth has been posing a
plethora of serious challenges such as climate change, enhanced greenhouse gases (GHG)
emissions, lack of appropriate infrastructure, traffic congestion, and lack of basic amenities
(electricity, water, and sanitation) in many localities, etc. (Ramachandra and Bharath 2016).
Peenya Industrial estate (PIE), Bangalore South Region (BSR) and Whitefield area (WF) are
considered (Fig. 1) to understand the driving forces of urban growth at micro levels. PIE is one
of the oldest and largest industrial areas in south-east Asia with an area of 922 ha,
established in 1977 by the Karnataka State Small Industries Development Corporation (KSSIDC) in
two stages with an annual turnover of around Rs. 110,000 million. The industrial estate houses
small, medium, as well as large scale industries and it lies between Bangalore-Mangalore Highway
(NH-48) and Bangalore- Mumbai (NH-4), convenient for transportation. BSR with an area of 3422 ha
is primarily dominated by residential, commercial complexes and IT companies, located on
Bannerghatta road connecting outer ring road. Whitefield region has developed with an intention
to attract major global technology players, a number of multinational information technology
(IT) companies. Until the late 1980s, WF was a small village with a retirement colony of
Anglo-Indians covering an area of 2205 ha. The Export Promotion Industrial Park (EPIP) is one of
the country's first information technology parks - (ITPB), which houses offices of many IT and
ITES companies. The residential constructions were started later 1990s and especially during
2002 onwards more apartment complexes have come. The field investigations were carried out to
understand the growth, type of growth and consequences on the local environment at these
locations.
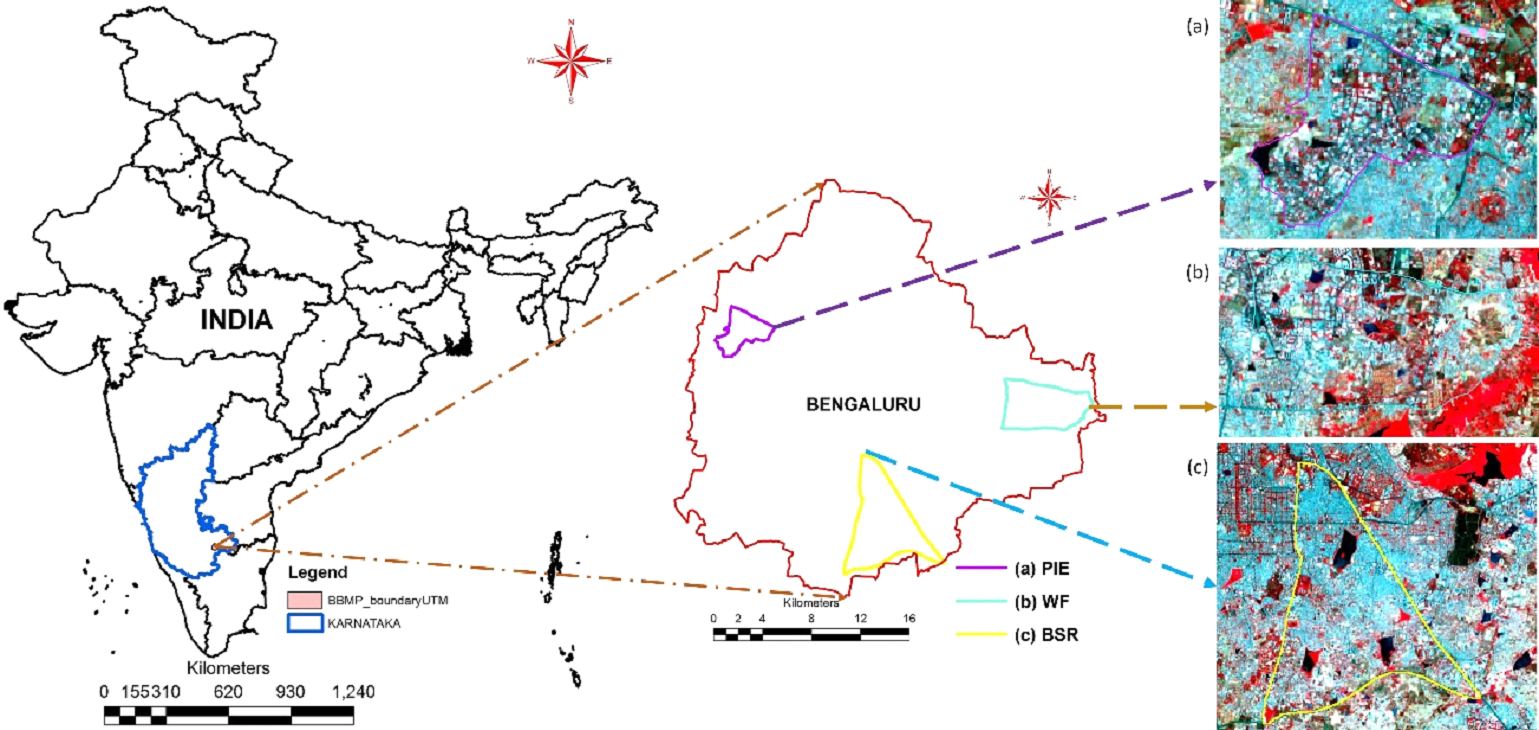
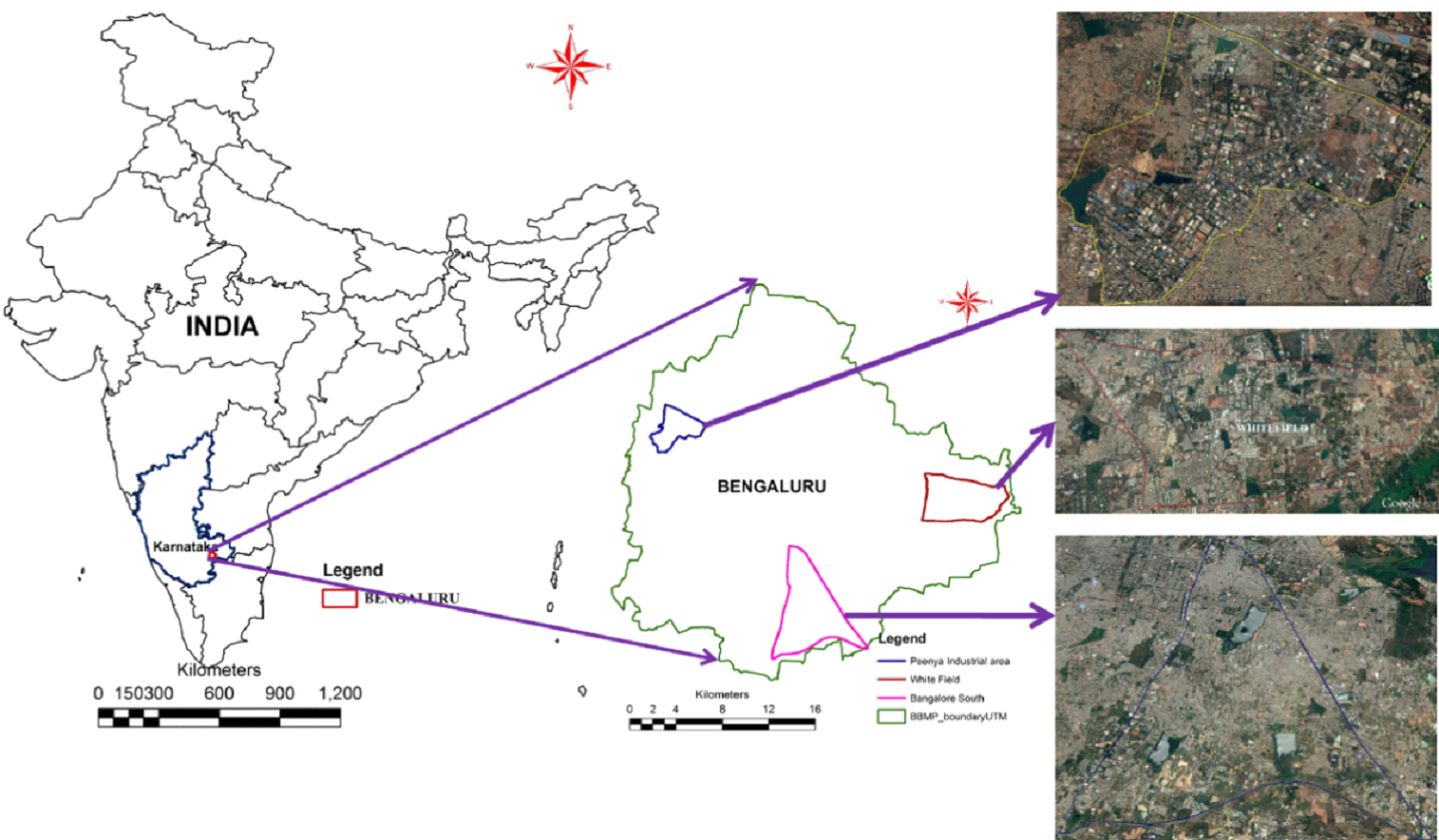
Fig. 1 Bangalore and the major growth centers – (a) Peenya Industrial estate [PIE], (b) Whitefield area [WF], (c) Bangalore South Region [BSR]
Method
Fig. 2 outlines the method adopted for understanding land use dynamics, which involved – (i) data collection and image pre-processing, (ii) land use analysis and quantification of spatial metrics, (iii) modelling and visualisation.
Data collection and image pre-processing: The primary data (Remote Sensing) includes multi temporal Landsat 1-MSS (1973), Landsat 5-TM (1992, 2003, 2008), Landsat 7-ETM+ (2012), Landsat 8-OLI (2017) and Google Earth (http://earth.google.com). Landsat data is a cost-effective and available free for downloading from public domains such as USGS (http://glovis.usgs.gov, http://earthexplorer.usgs.gov). Survey of India (SOI) topo-sheets of 1:50000 and 1:250000 scales (http://www.thesurveyofindia.gov.in) were used to generate base layers of the boundary, etc. Ground control points collected from the field using pre-calibrated handheld GPS (Global Positioning System), online spatial data portals Bhuvan (http://bhuvan.nrsc.gov.in) and Google Earth (http://earth.google.com) are used for geometric correction of remote sensing data. Then geo-corrected data was resampled to 30 m to maintain uniform resolutions across multiple datasets.
Land Use Analysis and quantification of spatial metrics: Land use analysis involved (i) creation of FCC (False color composite) by using multispectral bands (ii) training polygons were selected by locating heterogeneous patches on the FCC. These training polygons are distributed uniformly across the region (iii) 60% of polygons converted into signatures and land use classification has been carried out by using supervised Gaussian maximum likelihood classification algorithm using GRASS GIS. This classifier has been considered as one of the most superior methods which perform classification on the basis of probability density function (Bharath et al. 2013b; Ramachandra et al. 2018a). (iv) 40 % of polygons are used for accessing accuracy through Kappa statistics. Spatial metrics are a series of quantitative indices analysed using FRAGSTAT 3.3 (https://www.umass.edu/landeco/research/fragstats/fragstats.html). Table 1 lists the prioritized spatial metrics and their significance chosen for assessing spatiotemporal patterns of urbanization (Herold et al. 2005, Uuemaa et al. 2009; Aguilera, et al. 2011, Bharath et al. 2012; Ramachandra et al. 2012b; Bharath et al. 2017) at these locations.
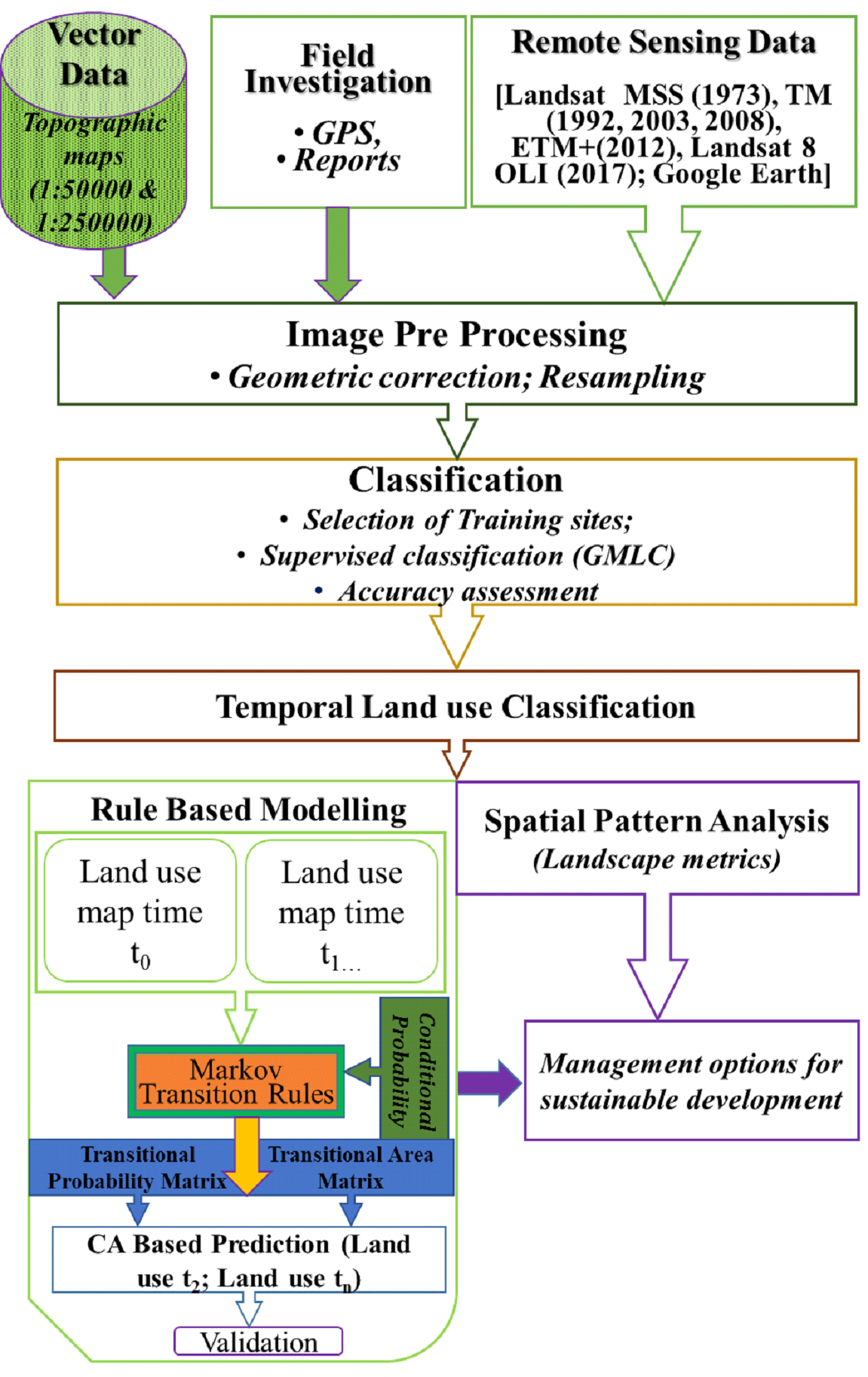
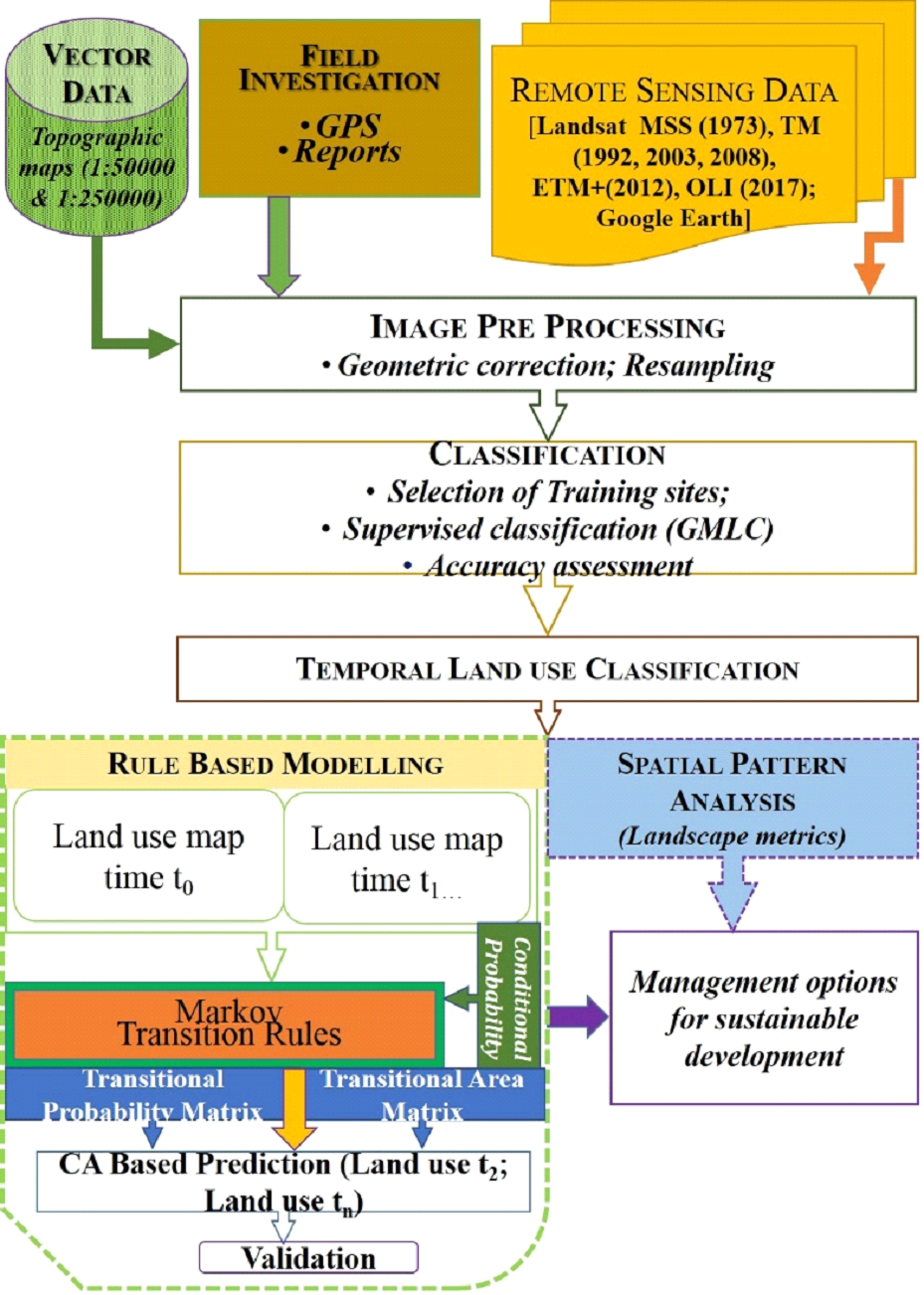
Fig. 2 Method adopted for urbanisation analysis.
Table 1 Landscape metrics analysed and their description.
Sno |
Indicators |
Formula |
Range |
Significance |
1 |
Class Area (CA) |
A LU = Area of land use A= Total landscape area |
>0 |
It represents the area covered by each land use feature to the total landscape area. |
2 |
Number of Patches (Built-up) |
NP equals the number of built-up patches in the landscape. |
NP>0, without limit |
It is a fragmentation Index. Higher the value more the fragmentation |
3 |
Largest Patch Index (Percentage of built-up) |
a i = area (m2) of patch i A= total landscape area |
0 ≤ LPI≤100 |
LPI = 0 when largest patch of the patch type becomes increasingly smaller. LPI = 100 when the entire landscape consists of a single patch comprise 100% of the landscape. |
4 |
Area Weighted Mean Patch Fractal Dimension (AWMPFD) |
where si and pi are the area and perimeter of patch i, and N is the total number of patches |
1≤AWMPFD≤2 |
AWMPFD approaches 1 for shapes with very simple perimeters, such as circles or squares, and approaches 2 for shapes with highly convoluted perimeter. |
5 |
Ratio of Open Space (ROS) |
where s is the summarization area of all “holes” inside the extracted urban area, s is summarization area of all patches |
Represented as percentage |
The ratio, in a development of open space to developed land. |
Modelling and Visualisation: Markov approach has provided information about transition probability between two LU classes with respect to time (t to time t+1) and transitional area matrix with respect to likely land use changes (extent) of each class. Transitional probability matrix and area matrix are obtained by equations 1 and 2.
where P is the Transitional probability matrix; Pij is the probability of ith land use to convert into jth class during the transition period; n is the number of land use classes.
Transition area matrix is obtained by (2) where A is the Transitional area matrix; Pij is the sum of the area of ith land use to convert into jth class during the transition period.
Cellular Automata (CA) aided in simulating and predicting land use changes based on the transitional rules depending on the state of cell changes according to the neighbourhood cells and the previous state of the current cell with the local and regional interactions. CA-Markov incorporates the transitional rules and probabilities collectively and provides better results. The land use for the future can be predicted by equation 3.
where L (t+1) is the land use status at time t+1; L (t) is the land use status at time t.
A contiguity filter of kernel size 55 was used that accounts for the neighbouring pixels. Land use maps of 2003, 2008 and 2012 were used for estimating transition probability and area matrices through Markov model. The transition probability matrices of three gradients depict transition of vegetation and others class to built-up (Table 2). The diagonal values represent the persistence of the class from 2012 to 2017. Then, Markov-CA model was used to simulate land uses of 2012 and 2017, which was compared with the actual land uses, and the accuracy of the simulation is assessed through Kappa statistics. The traditional Kappa statistics lacks in assessing prediction error with respect to pixel location (Pontius and Millones 2011). The revised kappa statistics were assessed to understand the accuracies of the prediction as compared to traditional kappa (Kstandard). A revised general kappa defined as kappa for no ability (Kno), Kquantity and Klocation. The Kquantity and Klocation are able to distinguish clearly between quantification error and location error, respectively (Pontius 2000; Ahmed et al. 2013). After successful validation, land use for the year 2022 is predicted with the aid of actual land uses of 2012-2017.
Table 2 Transition probability matrices of three gradients from 2012 to 2017
Gradient🡪 |
PIE |
WF |
BSR |
|||||||||
2012🡪 2017 |
BU |
V |
W |
O |
BU |
V |
W |
O |
BU |
V |
W |
O |
Built-up (BU) |
0.89 |
0.111 |
0.000 |
0.000 |
0.85 |
0.05 |
0.05 |
0.05 |
0.85 |
0.050 |
0.05 |
0.05 |
Vegetation (V) |
0.9 |
0.103 |
0.00 |
0.00 |
0.861 |
0.139 |
0.00 |
0.00 |
0.948 |
0.052 |
0.00 |
0.00 |
Water (W) |
0.12 |
0.00 |
0.88 |
0.000 |
0.173 |
0.014 |
0.813 |
0.00 |
0.184 |
0.00 |
0.816 |
0.00 |
Others (O) |
0.62 |
0.004 |
0.00 |
0.382 |
0.461 |
0.016 |
0.00 |
0.523 |
0.272 |
0.009 |
0.00 |
0.718 |